filmov
tv
BERT: Pre-training of Deep Bidirectional Transformers for Language Understanding
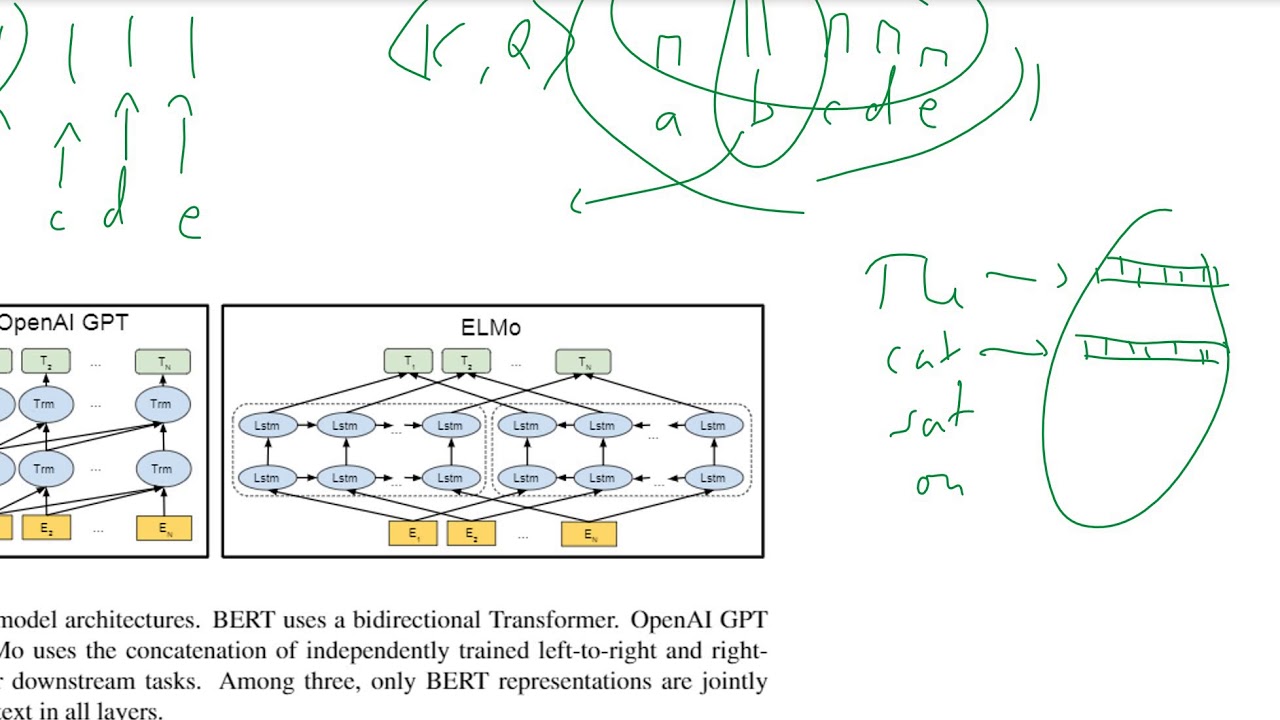
Показать описание
Abstract:
We introduce a new language representation model called BERT, which stands for Bidirectional Encoder Representations from Transformers. Unlike recent language representation models, BERT is designed to pre-train deep bidirectional representations by jointly conditioning on both left and right context in all layers. As a result, the pre-trained BERT representations can be fine-tuned with just one additional output layer to create state-of-the-art models for a wide range of tasks, such as question answering and language inference, without substantial task-specific architecture modifications.
BERT is conceptually simple and empirically powerful. It obtains new state-of-the-art results on eleven natural language processing tasks, including pushing the GLUE benchmark to 80.4% (7.6% absolute improvement), MultiNLI accuracy to 86.7 (5.6% absolute improvement) and the SQuAD v1.1 question answering Test F1 to 93.2 (1.5% absolute improvement), outperforming human performance by 2.0%.
Authors:
Jacob Devlin, Ming-Wei Chang, Kenton Lee, Kristina Toutanova
Комментарии