filmov
tv
Robust Source-Free Domain Adaptation for Fundus Image Segmentation
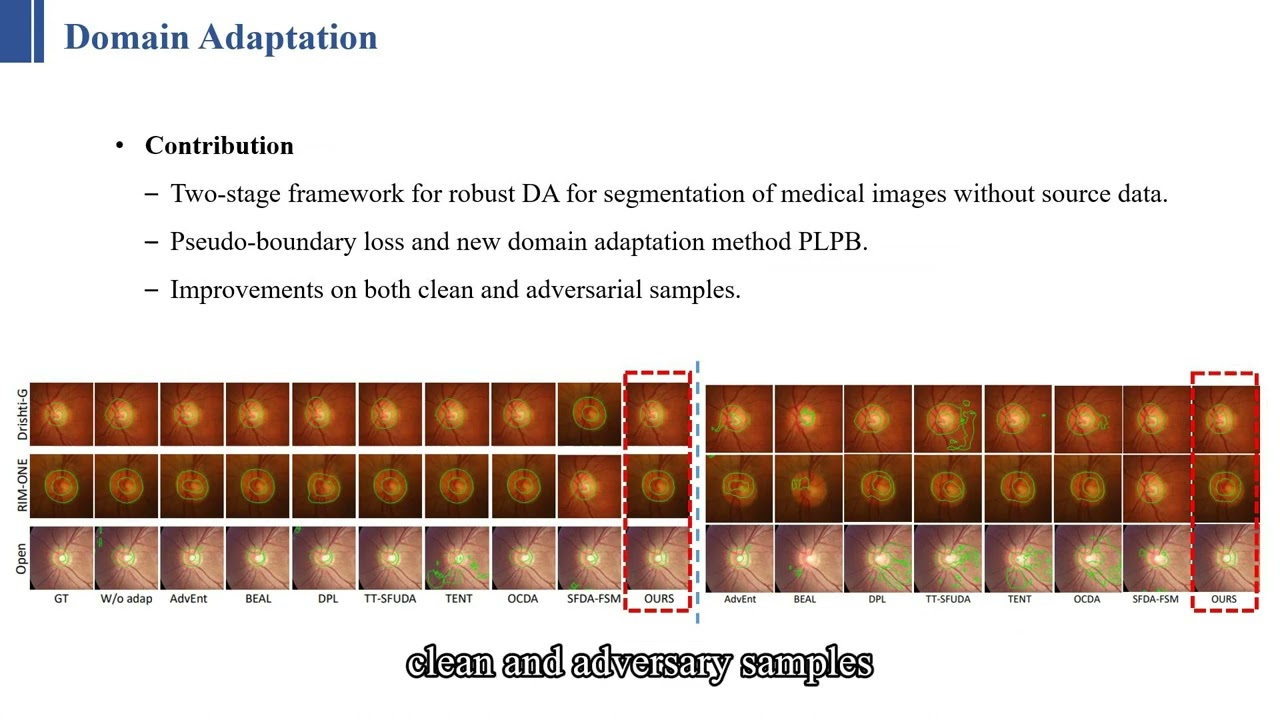
Показать описание
Authors: Lingrui Li; Yanfeng Zhou; Ge Yang
Description: Unsupervised Domain Adaptation (UDA) is a learning technique that transfers knowledge learned in the source domain from labelled training data to the target domain with only unlabelled data. It is of significant importance to medical image segmentation because of the usual lack of labelled training data. Although extensive efforts have
been made to optimize UDA techniques to improve the accuracy of segmentation models in the target domain, few studies have addressed the robustness of these models under UDA. In this study, we propose a two-stage training strategy for robust domain adaptation. In the source training stage, we utilize adversarial sample augmentation to enhance the robustness and generalization capability of the source model. And in the target training stage, we propose a novel robust pseudo-label and pseudo-boundary (PLPB)
Description: Unsupervised Domain Adaptation (UDA) is a learning technique that transfers knowledge learned in the source domain from labelled training data to the target domain with only unlabelled data. It is of significant importance to medical image segmentation because of the usual lack of labelled training data. Although extensive efforts have
been made to optimize UDA techniques to improve the accuracy of segmentation models in the target domain, few studies have addressed the robustness of these models under UDA. In this study, we propose a two-stage training strategy for robust domain adaptation. In the source training stage, we utilize adversarial sample augmentation to enhance the robustness and generalization capability of the source model. And in the target training stage, we propose a novel robust pseudo-label and pseudo-boundary (PLPB)