filmov
tv
Reproducibility, Reusability, & Robustness in Deep Reinforcement Learning - Prof. Pineau
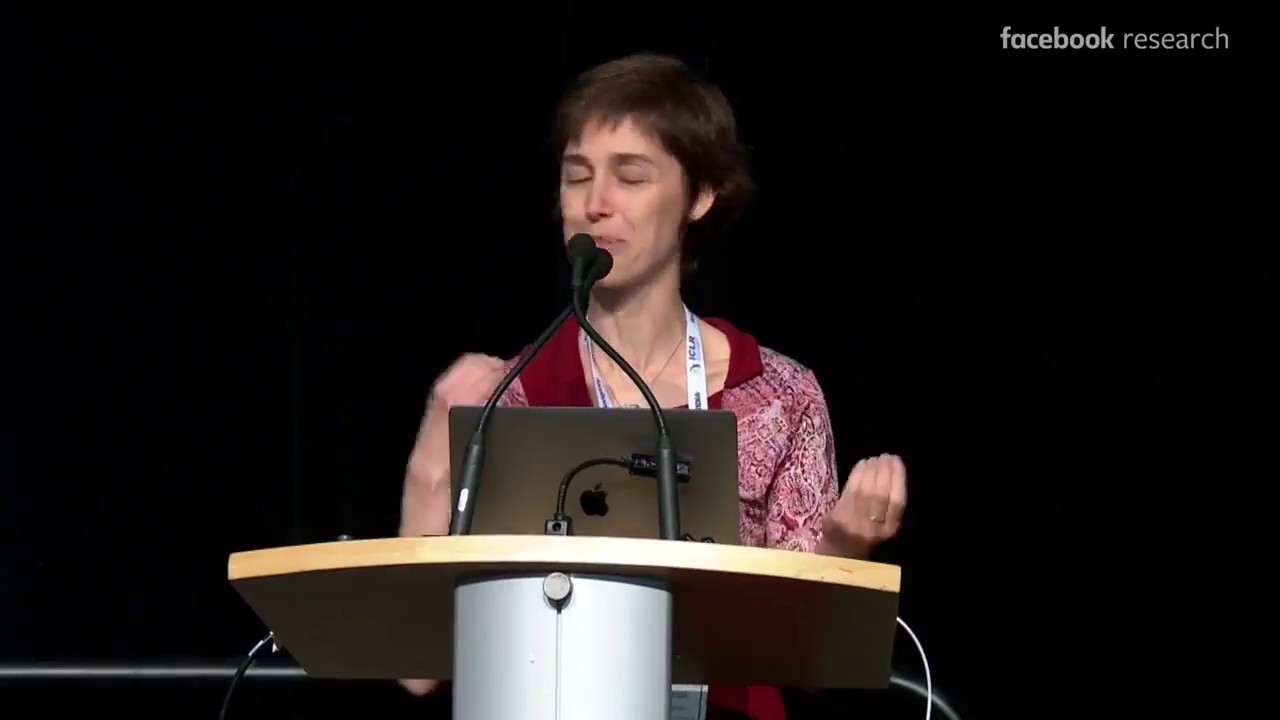
Показать описание
Recorded May 3rd, 2018
"In recent years, significant progress has been made in solving challenging problems across various domains using deep reinforcement learning. However reproducing results for state-of-the-art deep RL methods is seldom straightforward. High variance of some methods can make learning particularly difficult when environments or rewards are strongly stochastic. Furthermore, results can be brittle to even minor perturbations in the domain or experimental procedure. In this talk, I will discuss challenges that arise in experimental techniques and reporting procedures in deep RL, and will suggest methods and guidelines to make future results more reproducible, reusable and robust. I will also report on findings from the ICLR 2018 reproducibility challenge." - Prof. Joelle Pineau
"In recent years, significant progress has been made in solving challenging problems across various domains using deep reinforcement learning. However reproducing results for state-of-the-art deep RL methods is seldom straightforward. High variance of some methods can make learning particularly difficult when environments or rewards are strongly stochastic. Furthermore, results can be brittle to even minor perturbations in the domain or experimental procedure. In this talk, I will discuss challenges that arise in experimental techniques and reporting procedures in deep RL, and will suggest methods and guidelines to make future results more reproducible, reusable and robust. I will also report on findings from the ICLR 2018 reproducibility challenge." - Prof. Joelle Pineau
Reproducibility, Reusability, & Robustness in Deep Reinforcement Learning - Prof. Pineau
Joelle Pineau: Reproducibility, Reusability, and Robustness in Deep Reinforcement Learning ICLR 2018
15. Reproducibility, Reusability, And Robustness In Deep Reinforcement Learning
Dr. Joelle Pineau - Reproducible, Reusable, and Robust Reinforcement Learning - NeurIPS 2018
Reproducible, Reusable, and Robust Reinforcement Learning -Joelle Pineau
Reproducibility in Data Science | NYU
Building Reproducible, Reusable & Robust Deep Reinforcement Learning Systems | Jöelle Pineau
MLOps: From notebooks to reproducibility and reusability (Part 1: ML Development and experiments)
ACM DEBS 2020: Building Reproducible Reusable and Robust Machine Learning Software (Joelle Pineau)
Reproducibility, Replicability, and Robustness: J. Flanagan LADAL Opening Webinar Series 2021
Webinar: Best Practices for Reproducible, Collaborative Data Science
TechAide AI4Good 2020 - Joelle Pineau: Building Reproducible, Reusable and Robust Deep RL Systems
Reusedata: An Open-Source, Open-Development Tool For Reusable & Reproducible Genomic Data Manage...
Enable Reproducible, Scalable, and Orchestrated Workflows in HPC and Cloud-Converged Infrastructure
Week 4 - Reproducibility in Machine Learning: From Theory to Practice - Koustuv Sinha
Reproducibility in High Performance Computing
Open Science, Reuse, and Reproducibility with Flywheel Webinar
Implementing Increased Transparency and Reproducibility in Economics — Lars Vilhuber
Aidan Heerdegen: RRR: Reliability, Replicability, Reproducibility for climate models
Checking a reproducible data analysis using make, git, and R: Testing with new data! (CC075)
Reproducible and Open Data Science
METASCIENCE 2021 9 23 Building a Research Culture for Replicability and Reproducibility in the...
Implementing Increased Transparency and Reproducibility in Economics
Is AI Facing A Reproducibility Crisis?
Комментарии