filmov
tv
Austin Deep Learning Meetup - Llama 3 Candidate Paper | Self-Rewarding Language Models
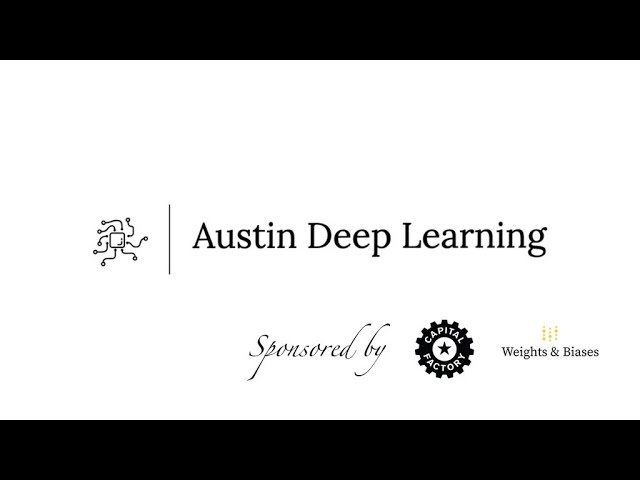
Показать описание
This will be a journal club event
Self-Rewarding Language Models by Yuan, Weizhe., et. al. (2024) Meta and NYU
Please print out or have the paper on your device.
Speaker
Dmitri Iourovitski
Abstract
We posit that to achieve superhuman agents, future models require super- human feedback in order to provide an adequate training signal. Current approaches commonly train reward models from human preferences, which may then be bottlenecked by human performance level, and secondly these separate frozen reward models cannot then learn to improve during LLM training.
In this work, we study Self-Rewarding Language Models, where the language model itself is used via LLM-as-a-Judge prompting to provide its own rewards during training.
We show that during Iterative DPO training that not only does instruction following ability improve, but also the ability to provide high-quality rewards to itself. Fine-tuning Llama 2 70B on three iterations of our approach yields a model that outperforms many existing systems on the AlpacaEval 2.0 leaderboard, including Claude 2, Gemini Pro, and GPT-4 0613. While only a preliminary study, this work opens the door to the possibility of models that can continually improve in both axes.
Info
Spots are limited to keep the discussions organized.
Austin Deep Learning Journal Club is group for committed machine learning practitioners and researchers alike. The group typically meets every first Tuesday of each month to discuss research publications. The publications are usually the ones that laid foundation to ML/DL or explore novel promising ideas and are selected by a vote. Participant are expected to read the publications to be able to contribute to discussion and learn from others. This is also a great opportunity to showcase your implementations to get feedback from other experts.
Sponsors:
Capital Factory (Austin, Texas)
Self-Rewarding Language Models by Yuan, Weizhe., et. al. (2024) Meta and NYU
Please print out or have the paper on your device.
Speaker
Dmitri Iourovitski
Abstract
We posit that to achieve superhuman agents, future models require super- human feedback in order to provide an adequate training signal. Current approaches commonly train reward models from human preferences, which may then be bottlenecked by human performance level, and secondly these separate frozen reward models cannot then learn to improve during LLM training.
In this work, we study Self-Rewarding Language Models, where the language model itself is used via LLM-as-a-Judge prompting to provide its own rewards during training.
We show that during Iterative DPO training that not only does instruction following ability improve, but also the ability to provide high-quality rewards to itself. Fine-tuning Llama 2 70B on three iterations of our approach yields a model that outperforms many existing systems on the AlpacaEval 2.0 leaderboard, including Claude 2, Gemini Pro, and GPT-4 0613. While only a preliminary study, this work opens the door to the possibility of models that can continually improve in both axes.
Info
Spots are limited to keep the discussions organized.
Austin Deep Learning Journal Club is group for committed machine learning practitioners and researchers alike. The group typically meets every first Tuesday of each month to discuss research publications. The publications are usually the ones that laid foundation to ML/DL or explore novel promising ideas and are selected by a vote. Participant are expected to read the publications to be able to contribute to discussion and learn from others. This is also a great opportunity to showcase your implementations to get feedback from other experts.
Sponsors:
Capital Factory (Austin, Texas)