filmov
tv
10M Token Context Windows vs RAG: Do You Still Need Retrieval?
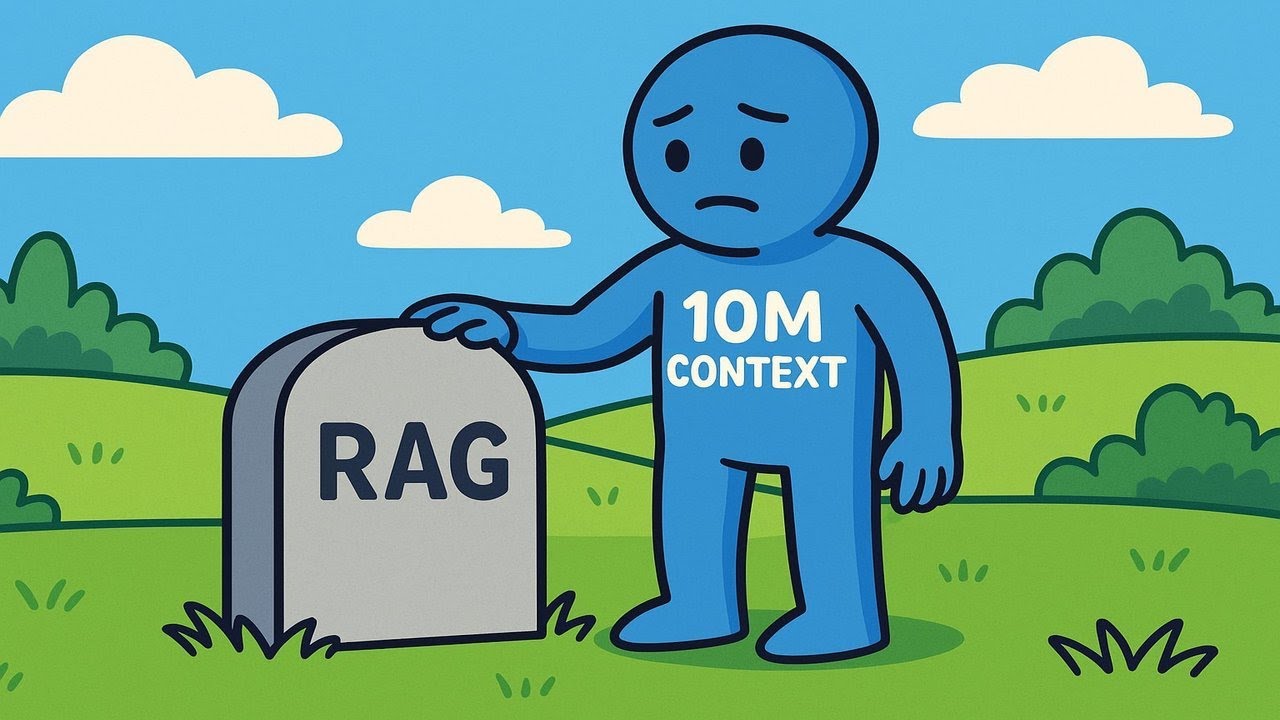
Показать описание
10M Token Context Windows vs RAG: Do You Still Need Retrieval?
In this video, I break down a common myth in the AI world:
Does a 10 million token context window eliminate the need for RAG (Retrieval-Augmented Generation)?
Spoiler: It doesn’t.
Yes, massive context windows—like the one in Meta’s LLaMA 4—are a huge advancement in large language model capabilities. But that doesn't mean you should throw out RAG. In fact, RAG becomes even more important as your dataset scales, especially in enterprise settings.
🔍 What you’ll learn in this video:
What a 10M token context window actually means (in real-world terms)
Why context size doesn’t replace smart retrieval
How I use RAG with my own tweet history to keep prompts efficient and relevant
Why enterprise AI still needs RAG to scale
💡 Perfect for: AI engineers, architects, and enterprise IT leaders building AI-native applications with LLMs like GPT-4, Claude, and LLaMA.
📌 Keywords:
10M token context window, LLaMA 4, RAG, retrieval augmented generation, large language models, enterprise AI, vector search, prompt engineering, context window vs retrieval, AI infrastructure, GPT-4, LLM architecture
In this video, I break down a common myth in the AI world:
Does a 10 million token context window eliminate the need for RAG (Retrieval-Augmented Generation)?
Spoiler: It doesn’t.
Yes, massive context windows—like the one in Meta’s LLaMA 4—are a huge advancement in large language model capabilities. But that doesn't mean you should throw out RAG. In fact, RAG becomes even more important as your dataset scales, especially in enterprise settings.
🔍 What you’ll learn in this video:
What a 10M token context window actually means (in real-world terms)
Why context size doesn’t replace smart retrieval
How I use RAG with my own tweet history to keep prompts efficient and relevant
Why enterprise AI still needs RAG to scale
💡 Perfect for: AI engineers, architects, and enterprise IT leaders building AI-native applications with LLMs like GPT-4, Claude, and LLaMA.
📌 Keywords:
10M token context window, LLaMA 4, RAG, retrieval augmented generation, large language models, enterprise AI, vector search, prompt engineering, context window vs retrieval, AI infrastructure, GPT-4, LLM architecture