filmov
tv
Subhojeet Pramanik - AGaLiTe: Approx. Gated Linear Transformers for Online Reinforcement Learning
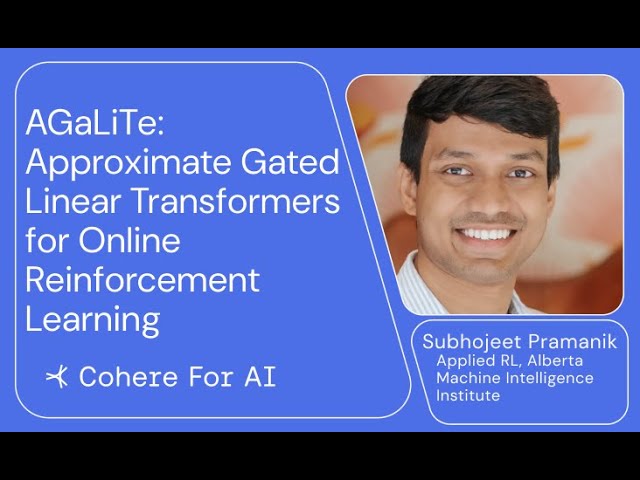
Показать описание
In this paper we investigate transformer architectures designed for partially observable online reinforcement learning. The self-attention mechanism in the transformer architecture is capable of capturing long-range dependencies and it is the main reason behind its effectiveness in processing sequential data. Nevertheless, despite their success, transformers have two significant drawbacks that still limit their applicability in online reinforcement learning: (1) in order to remember all past information, the self-attention mechanism requires access to the whole history to be provided as context. (2) The inference cost in transformers is expensive. In this paper, we introduce recurrent alternatives to the transformer self-attention mechanism that offer context-independent inference cost, leverage long-range dependencies effectively, and performs well in online reinforcement learning task. We quantify the impact of the different components of our architecture in a diagnostic environment and assess performance gains in 2D and 3D pixel-based partially-observable environments (e.g. T-Maze, Mystery Path, Craftax, and Memory Maze). Compared with a state-of-the-art architecture, GTrXL, inference in our approach is at least 40% cheaper while reducing memory use more than 50%. Our approach either performs similarly or better than GTrXL, improving more than 37% upon GTrXL performance in harder tasks.
Subho specializes in deep learning and reinforcement learning. He earned his Master's degree from the RLAI lab at the University of Alberta, focusing on continual reinforcement learning. He has more than four years of experience in researching transformer models and applying them in various areas such as reinforcement learning, language modeling, multi-modal learning, and computer vision. He has worked in research and industry roles at companies like IBM, Huawei, and Alberta Machine Intelligence Institute (Amii), and co-founded a startup in the AI field. Currently, he is working as an ML Resident at Amii, applying continual reinforcement learning to outdoor active noise cancellation.
This session is brought to you by the Cohere For AI Open Science Community - a space where ML researchers, engineers, linguists, social scientists, and lifelong learners connect and collaborate with each other. Thank you to our Community Leads for organizing and hosting this event.
Subho specializes in deep learning and reinforcement learning. He earned his Master's degree from the RLAI lab at the University of Alberta, focusing on continual reinforcement learning. He has more than four years of experience in researching transformer models and applying them in various areas such as reinforcement learning, language modeling, multi-modal learning, and computer vision. He has worked in research and industry roles at companies like IBM, Huawei, and Alberta Machine Intelligence Institute (Amii), and co-founded a startup in the AI field. Currently, he is working as an ML Resident at Amii, applying continual reinforcement learning to outdoor active noise cancellation.
This session is brought to you by the Cohere For AI Open Science Community - a space where ML researchers, engineers, linguists, social scientists, and lifelong learners connect and collaborate with each other. Thank you to our Community Leads for organizing and hosting this event.