filmov
tv
Machine Learning in Robotics - Imitation Learning for Soft Robots - Matthew Howard
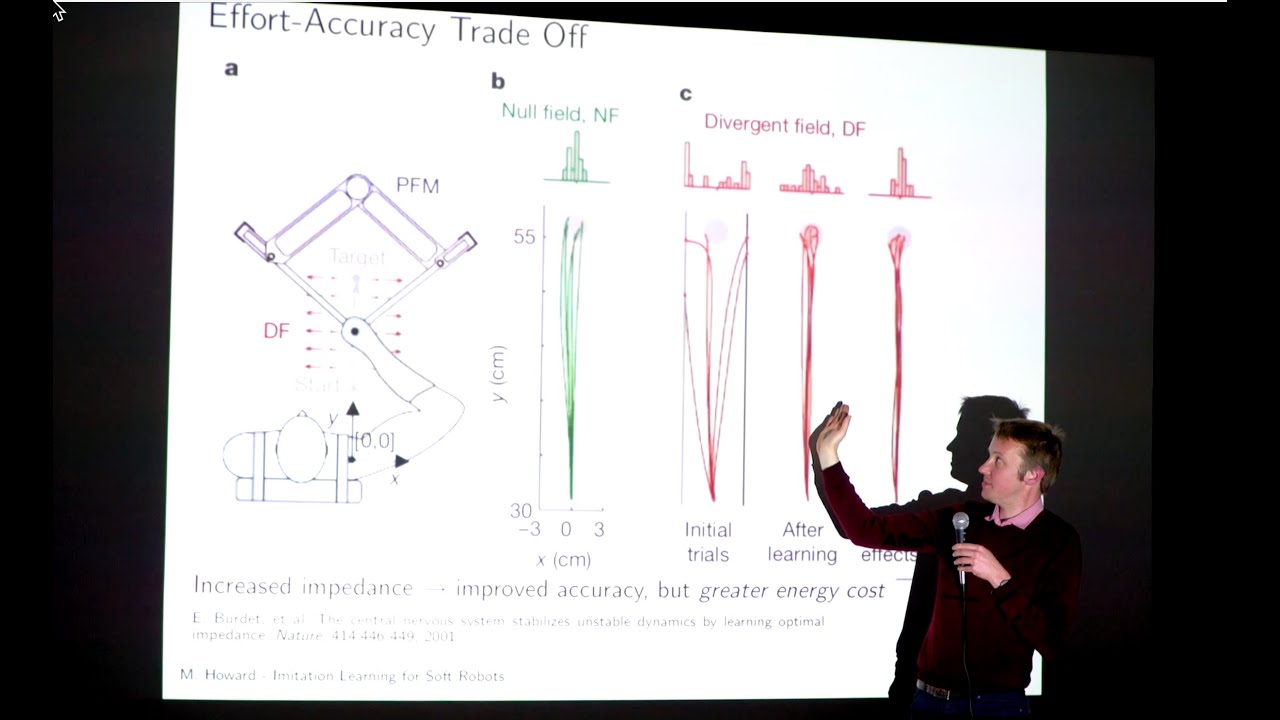
Показать описание
This is a recording of the seminar held by London Machine Learning Meetup group on 23rd May 2016.
Imitation Learning for Soft Robots - Matthew Howard (KCL)
Inspired by the antagonistic human musculoskeletal system, the current trend in mechatronic design is to include physically compliant elements into the embodiment of robotic devices. The promise of such ‘soft robotic’ systems, includes safety and agility. However, these offerings are tempered by the increased complexity of the system dynamics leading to difficulty in control. Learning (by demonstration or reinforcement) is often advocated as a means of dealing with this complexity, and can allow us to exploit the principles of human sensorimotor control to improve our robotic systems. In this talk, I will present work in programming soft robots through imitation of humans, both through forward and inverse optimal control, in order to exploit their dynamic characteristics, and talk about my view of up-and-coming topics in compliant robotics.
Bio: Dr Matthew Howard is a lecturer at the Centre for Robotics Research, Dept. Informatics, King's College London. Prior to joining King's in Summer 2013, he held a Japan Society for Promotion of Science fellowship at the Department of Mechanoinformatics at the University of Tokyo. From 2009-2012 he was a research fellow at the University of Edinburgh involved in the EU-FY7 STIFF project, and also obtained his PhD in 2009 at Edinburgh with award of an EPSRC CASE award, sponsored by Honda Research. He is internationally recognised in the fields of robotics and autonomous systems, statistical machine learning and adaptive control, with invitation to talk in leading conferences in these fields. His current interests include robotic skill learning and fast (re)programming by demonstration for soft robotic devices, design and control of variable impedance devices and EMG-based robot control and teleimpedance.
Imitation Learning for Soft Robots - Matthew Howard (KCL)
Inspired by the antagonistic human musculoskeletal system, the current trend in mechatronic design is to include physically compliant elements into the embodiment of robotic devices. The promise of such ‘soft robotic’ systems, includes safety and agility. However, these offerings are tempered by the increased complexity of the system dynamics leading to difficulty in control. Learning (by demonstration or reinforcement) is often advocated as a means of dealing with this complexity, and can allow us to exploit the principles of human sensorimotor control to improve our robotic systems. In this talk, I will present work in programming soft robots through imitation of humans, both through forward and inverse optimal control, in order to exploit their dynamic characteristics, and talk about my view of up-and-coming topics in compliant robotics.
Bio: Dr Matthew Howard is a lecturer at the Centre for Robotics Research, Dept. Informatics, King's College London. Prior to joining King's in Summer 2013, he held a Japan Society for Promotion of Science fellowship at the Department of Mechanoinformatics at the University of Tokyo. From 2009-2012 he was a research fellow at the University of Edinburgh involved in the EU-FY7 STIFF project, and also obtained his PhD in 2009 at Edinburgh with award of an EPSRC CASE award, sponsored by Honda Research. He is internationally recognised in the fields of robotics and autonomous systems, statistical machine learning and adaptive control, with invitation to talk in leading conferences in these fields. His current interests include robotic skill learning and fast (re)programming by demonstration for soft robotic devices, design and control of variable impedance devices and EMG-based robot control and teleimpedance.