filmov
tv
Interpretable Machine Learning
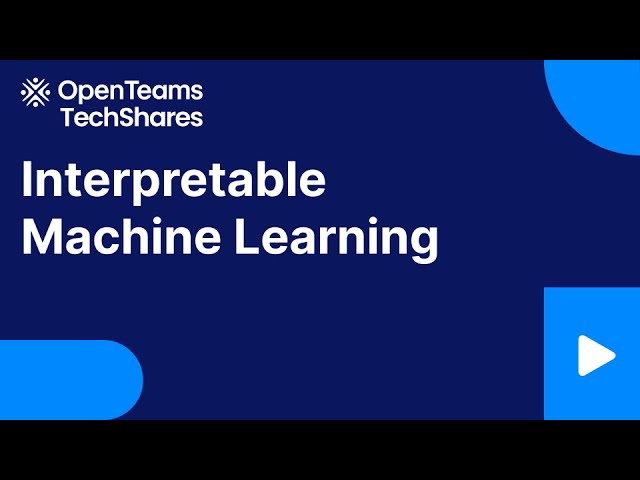
Показать описание
Machine Learning (ML) is quickly becoming ubiquitous in banking for both predictive analytics and process automation applications. However, banks in the US remain cautious in adopting ML for high risk and regulated areas such as credit underwriting. Among the key concerns are ML explainability and robustness. To address the inadequacy of post-hoc explainability tools in XAI for high stake applications, we developed inherently interpretable machine learning models including Deep ReLU networks and Functional ANOVA-based ML models. Model robustness is a key requirement as models will be subjected to constantly changing environments during production. A conceptually sound model must be able to function properly—without continuous retraining—and invariant under a changing environment. Recently, we released the PiML (Python Interpretable Machine Learning) package as a tool to design inherently interpretable models and to test machine learning robustness, reliability, and resilience.
Agus Sudjianto
Executive Vice President Head of Corporate Model Risk Wells Fargo & Company
Agus Sudjianto
Executive Vice President Head of Corporate Model Risk Wells Fargo & Company