filmov
tv
Generative Machine Learning Approaches for Data-Driven Modeling of Dynamics.
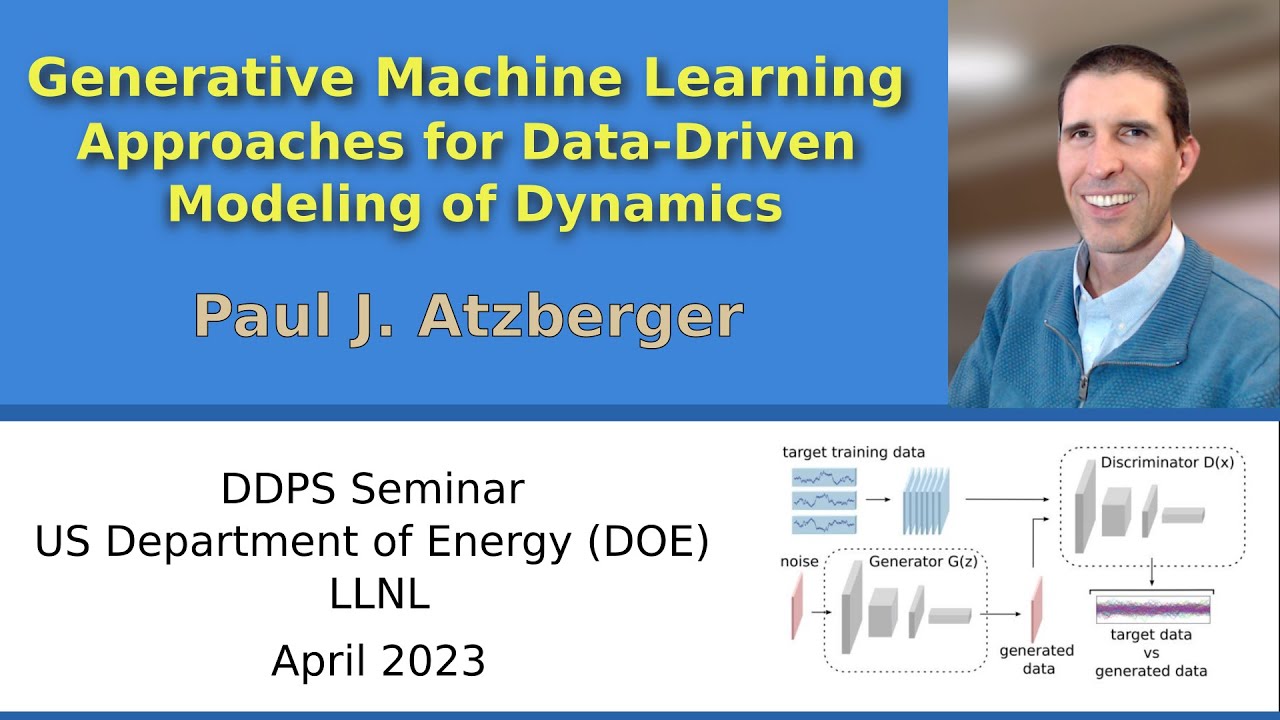
Показать описание
We discuss SDYN-GANs, adversarial learning, and related methods for learning representations, reductions, and unknown force laws for deterministic and stochastic systems. The work is motivated by applications in mechanics, coarse-grained modeling, and related problems arising in scientific simulations.
Talk in the DDPS Seminar at US Department of Energy (DOE) LLNL.
Related papers:
SDYN-GANs: Adversarial Learning Methods for Multistep Generative Models for General Order Stochastic Dynamics, P. Stinis, C. Daskalakis, and P. J. Atzberger, (arXiv), (2023),
GD-VAEs: Geometric Dynamic Variational Autoencoders for Learning Nonlinear Dynamics and Dimension Reductions, R. Lopez and P. J. Atzberger, (arXiv), (2022),
Related GD-VAE Talk:
Additional Talks
Additional information and software:
Talk in the DDPS Seminar at US Department of Energy (DOE) LLNL.
Related papers:
SDYN-GANs: Adversarial Learning Methods for Multistep Generative Models for General Order Stochastic Dynamics, P. Stinis, C. Daskalakis, and P. J. Atzberger, (arXiv), (2023),
GD-VAEs: Geometric Dynamic Variational Autoencoders for Learning Nonlinear Dynamics and Dimension Reductions, R. Lopez and P. J. Atzberger, (arXiv), (2022),
Related GD-VAE Talk:
Additional Talks
Additional information and software: