filmov
tv
How to Add a Zero at the Beginning of a Numpy Array: A Guide for Efficient Array Handling
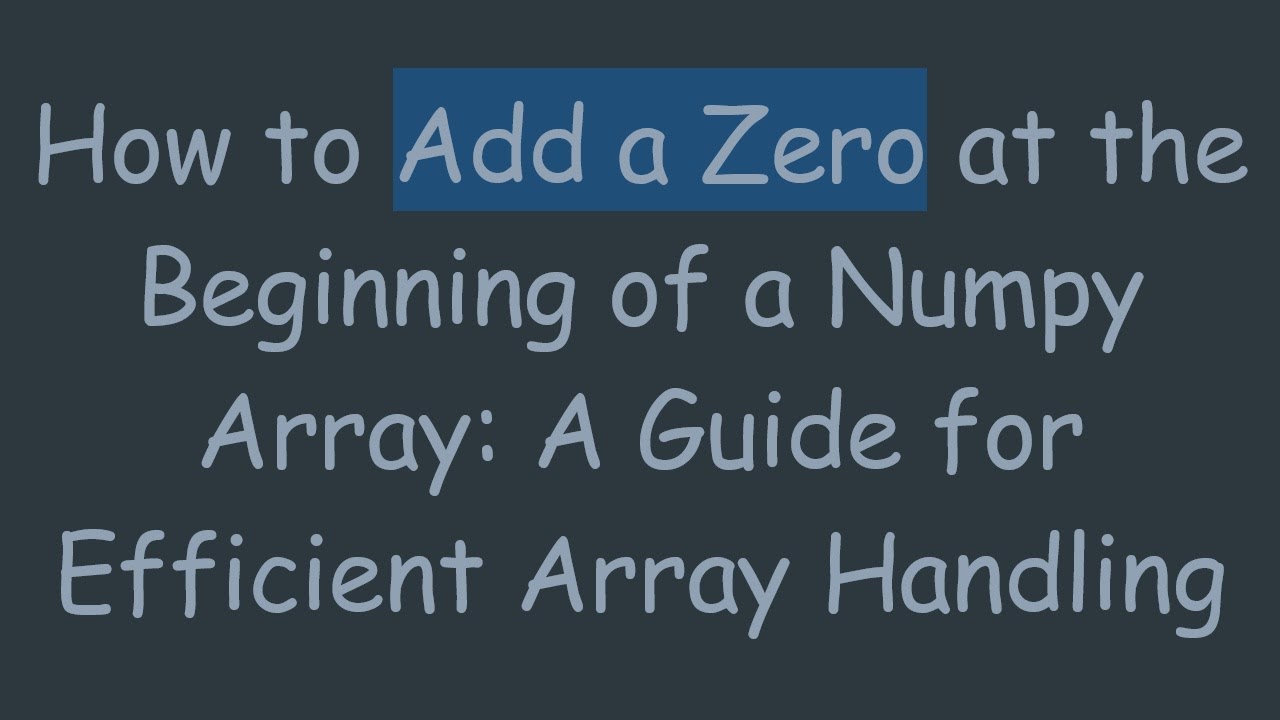
Показать описание
Discover a simple solution to add a leading zero in a Numpy array while optimizing memory usage. Learn how to implement it effectively!
---
Visit these links for original content and any more details, such as alternate solutions, latest updates/developments on topic, comments, revision history etc. For example, the original title of the Question was: adding a zero at first element in a numpy array or a way to create an array keeping the first position as zero
If anything seems off to you, please feel free to write me at vlogize [AT] gmail [DOT] com.
---
How to Add a Zero at the Beginning of a Numpy Array: A Guide for Efficient Array Handling
Numpy is an incredibly powerful library for numerical computing in Python. However, when working with arrays, especially for tasks like biological sequence analysis or any other string-related computation, you might face specific issues. For instance, you may need to create an array that starts with a zero, possibly for storing skew values in genomic sequences.
One common question that arises is, how can we insert a zero at the first position of a Numpy array? Let's explore this problem and its solution more systematically.
The Problem
Many users encounter a situation where they want to maintain the first position as zero when processing their data. This need often arises in applications like tracking genetic sequences.
Here’s a practical example from a user who designed a function to calculate a skew array based on the characters G and C in a given text:
[[See Video to Reveal this Text or Code Snippet]]
The Solution
Instead of appending zero at the end of the array after its creation, a more efficient way is to allocate an array that is one element longer from the start. This can save time and memory, making your function more efficient.
The Optimized Approach
Here’s the revised function:
[[See Video to Reveal this Text or Code Snippet]]
Key Changes Explained
Pre-allocation: The skew array is initialized to len(text) + 1 which guarantees that the first position (index 0) is naturally set to zero.
Dictionary for Lookups: By using a dictionary (skew_dict) to map characters to their corresponding values, we can avoid lengthy if-elif statements. This makes the function cleaner and potentially faster since dictionary lookups are generally faster than multiple conditional checks.
Starting the Enumeration: The enumerate function is configured to start from 1, which aligns perfectly with our array's indexing after the initial zero.
Benefits of This Method
Performance: Minimizing the number of times we change the size of the array can significantly improve the performance, especially with large datasets.
Memory Efficiency: Pre-allocating the array reduces the overhead associated with resizing arrays during execution, therefore using memory resources more effectively.
Readability: The code is cleaner and more maintainable, which is an essential factor in programming.
Conclusion
Creating a Numpy array with a leading zero doesn't have to be complicated. By following the structured approach above, you can ensure your implementations not only work correctly but also run efficiently. If you’re working on larger datasets or sequences, this method will save you time and resources, allowing you to focus on more critical aspects of your research or analysis.
Feel free to experiment with this function and adapt it to fit your specific requirements!
---
Visit these links for original content and any more details, such as alternate solutions, latest updates/developments on topic, comments, revision history etc. For example, the original title of the Question was: adding a zero at first element in a numpy array or a way to create an array keeping the first position as zero
If anything seems off to you, please feel free to write me at vlogize [AT] gmail [DOT] com.
---
How to Add a Zero at the Beginning of a Numpy Array: A Guide for Efficient Array Handling
Numpy is an incredibly powerful library for numerical computing in Python. However, when working with arrays, especially for tasks like biological sequence analysis or any other string-related computation, you might face specific issues. For instance, you may need to create an array that starts with a zero, possibly for storing skew values in genomic sequences.
One common question that arises is, how can we insert a zero at the first position of a Numpy array? Let's explore this problem and its solution more systematically.
The Problem
Many users encounter a situation where they want to maintain the first position as zero when processing their data. This need often arises in applications like tracking genetic sequences.
Here’s a practical example from a user who designed a function to calculate a skew array based on the characters G and C in a given text:
[[See Video to Reveal this Text or Code Snippet]]
The Solution
Instead of appending zero at the end of the array after its creation, a more efficient way is to allocate an array that is one element longer from the start. This can save time and memory, making your function more efficient.
The Optimized Approach
Here’s the revised function:
[[See Video to Reveal this Text or Code Snippet]]
Key Changes Explained
Pre-allocation: The skew array is initialized to len(text) + 1 which guarantees that the first position (index 0) is naturally set to zero.
Dictionary for Lookups: By using a dictionary (skew_dict) to map characters to their corresponding values, we can avoid lengthy if-elif statements. This makes the function cleaner and potentially faster since dictionary lookups are generally faster than multiple conditional checks.
Starting the Enumeration: The enumerate function is configured to start from 1, which aligns perfectly with our array's indexing after the initial zero.
Benefits of This Method
Performance: Minimizing the number of times we change the size of the array can significantly improve the performance, especially with large datasets.
Memory Efficiency: Pre-allocating the array reduces the overhead associated with resizing arrays during execution, therefore using memory resources more effectively.
Readability: The code is cleaner and more maintainable, which is an essential factor in programming.
Conclusion
Creating a Numpy array with a leading zero doesn't have to be complicated. By following the structured approach above, you can ensure your implementations not only work correctly but also run efficiently. If you’re working on larger datasets or sequences, this method will save you time and resources, allowing you to focus on more critical aspects of your research or analysis.
Feel free to experiment with this function and adapt it to fit your specific requirements!