filmov
tv
Building A Better Data Warehouse For The Cloud At Firebolt
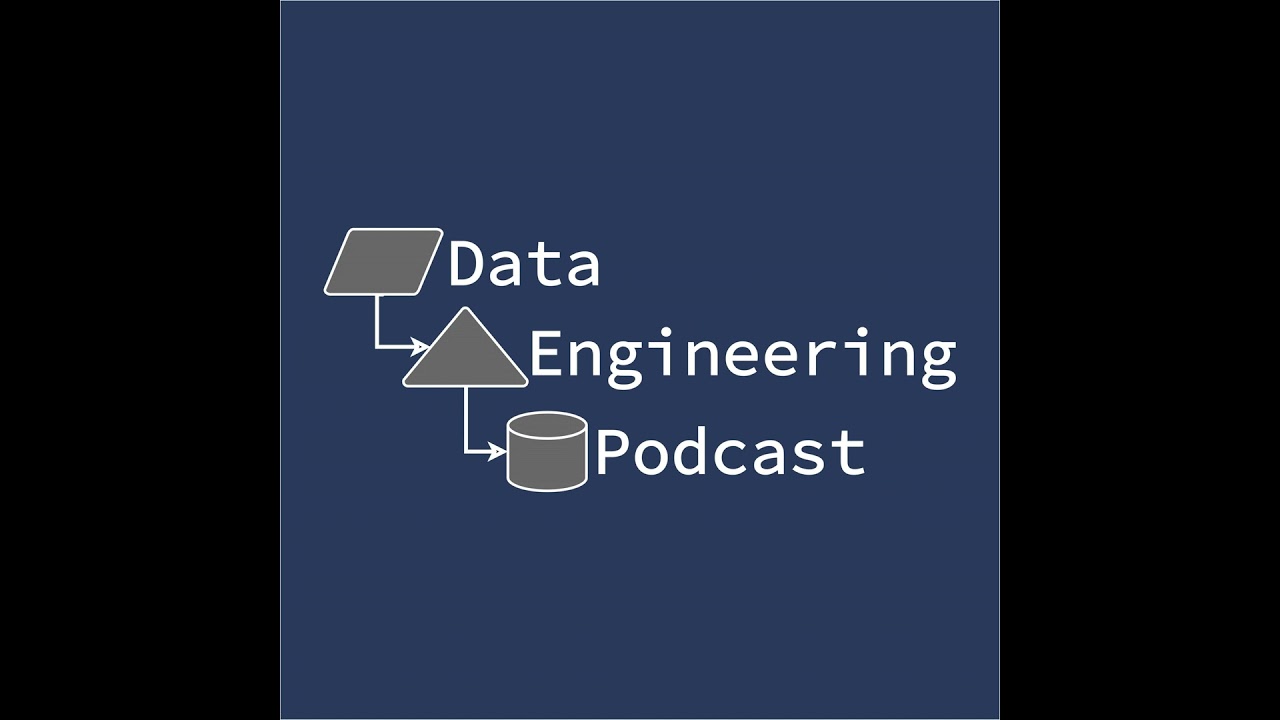
Показать описание
Summary
Data warehouse technology has been around for decades and has gone through several generational shifts in that time. The current trends in data warehousing are oriented around cloud native architectures that take advantage of dynamic scaling and the separation of compute and storage. Firebolt is taking that a step further with a core focus on speed and interactivity. In this episode CEO and founder Eldad Farkash explains how the Firebolt platform is architected for high throughput, their simple and transparent pricing model to encourage widespread use, and the use cases that it unlocks through interactive query speeds.
Announcements
• Hello and welcome to the Data Engineering Podcast, the show about modern data management
• Today’s episode of the Data Engineering Podcast is sponsored by Datadog, a SaaS-based monitoring and analytics platform for cloud-scale infrastructure, applications, logs, and more. Datadog uses machine-learning based algorithms to detect errors and anomalies across your entire stack—which reduces the time it takes to detect and address outages and helps promote collaboration between Data Engineering, Operations, and the rest of the company.
• Your host is Tobias Macey and today I’m interviewing Eldad Farkash about Firebolt, a cloud data warehouse optimized for speed and elasticity on structured and semi-structured data
Interview
• Introduction
• How did you get involved in the area of data management?
• Can you start by describing what Firebolt is and your motivation for building it?
• How does Firebolt compare to other data warehouse technologies what unique features does it provide?
• The lines between a data warehouse and a data lake have been blurring in recent years. Where on that continuum does Firebolt lie?
• What are the unique use cases that Firebolt allows for?
• How do the performance characteristics of Firebolt change the ways that an engineer should think about data modeling?
• What technologies might someone replace with Firebolt?
• How is Firebolt architected and how has the design evolved since you first began working on it?
• What are some of the most challenging aspects of building a data warehouse platform that is optimized for speed?
• How do you handle support for nested and semi-structured data?
• In what ways have you found it necessary/useful to extend SQL?
• Due to the immutability of object storage, for data lakes the update or delete process involves reprocessing a potentially large amount of data. How do you approach that in Firebolt with your F3 format?
• What have you found ...
Data warehouse technology has been around for decades and has gone through several generational shifts in that time. The current trends in data warehousing are oriented around cloud native architectures that take advantage of dynamic scaling and the separation of compute and storage. Firebolt is taking that a step further with a core focus on speed and interactivity. In this episode CEO and founder Eldad Farkash explains how the Firebolt platform is architected for high throughput, their simple and transparent pricing model to encourage widespread use, and the use cases that it unlocks through interactive query speeds.
Announcements
• Hello and welcome to the Data Engineering Podcast, the show about modern data management
• Today’s episode of the Data Engineering Podcast is sponsored by Datadog, a SaaS-based monitoring and analytics platform for cloud-scale infrastructure, applications, logs, and more. Datadog uses machine-learning based algorithms to detect errors and anomalies across your entire stack—which reduces the time it takes to detect and address outages and helps promote collaboration between Data Engineering, Operations, and the rest of the company.
• Your host is Tobias Macey and today I’m interviewing Eldad Farkash about Firebolt, a cloud data warehouse optimized for speed and elasticity on structured and semi-structured data
Interview
• Introduction
• How did you get involved in the area of data management?
• Can you start by describing what Firebolt is and your motivation for building it?
• How does Firebolt compare to other data warehouse technologies what unique features does it provide?
• The lines between a data warehouse and a data lake have been blurring in recent years. Where on that continuum does Firebolt lie?
• What are the unique use cases that Firebolt allows for?
• How do the performance characteristics of Firebolt change the ways that an engineer should think about data modeling?
• What technologies might someone replace with Firebolt?
• How is Firebolt architected and how has the design evolved since you first began working on it?
• What are some of the most challenging aspects of building a data warehouse platform that is optimized for speed?
• How do you handle support for nested and semi-structured data?
• In what ways have you found it necessary/useful to extend SQL?
• Due to the immutability of object storage, for data lakes the update or delete process involves reprocessing a potentially large amount of data. How do you approach that in Firebolt with your F3 format?
• What have you found ...