filmov
tv
Python for Machine Learning - Polynomial Linear Regression using Scikit Learn - P9
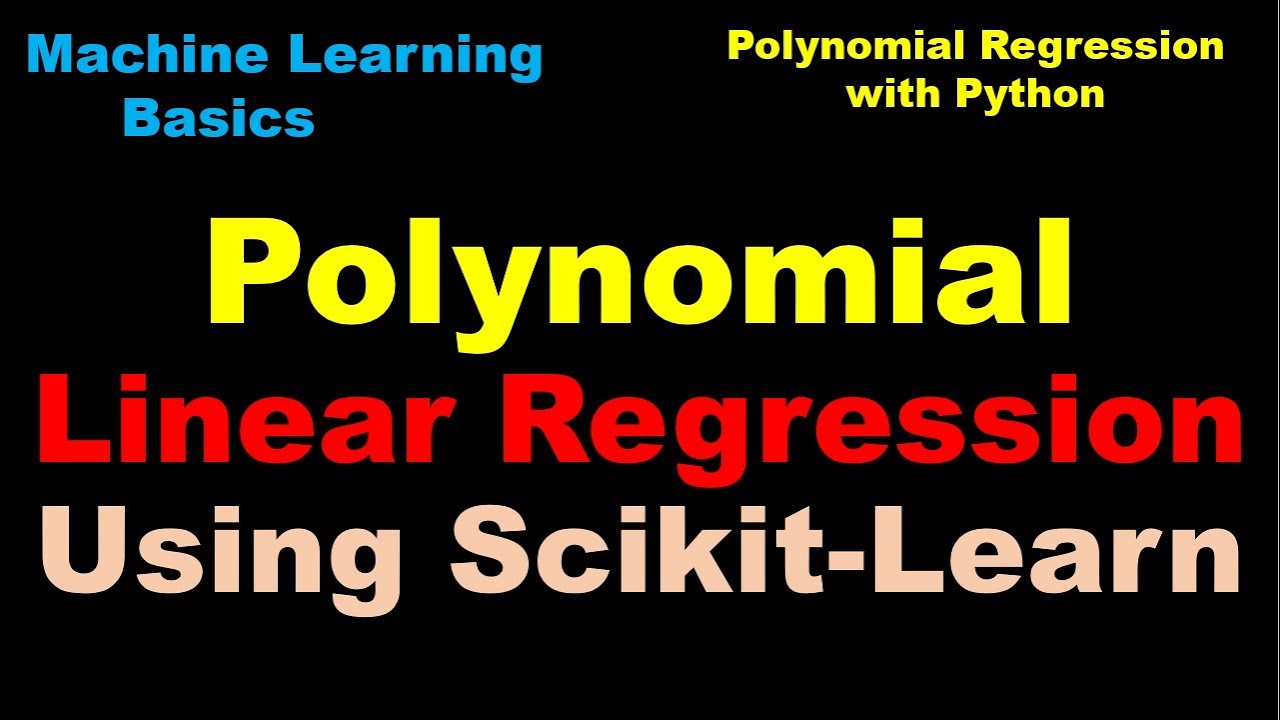
Показать описание
Python for Machine Learning - Polynomial Linear Regression using Scikit Learn - P9
Polynomial Linear Regression using Scikit Learn
In statistics, polynomial regression is a form of regression analysis in which the relationship between the independent variable x and the dependent variable y is modelled as an nth degree polynomial in x. Polynomial regression fits a nonlinear relationship between the value of x and the corresponding conditional mean of y, denoted E(y |x). Although polynomial regression fits a nonlinear model to the data, as a statistical estimation problem it is linear, in the sense that the regression function E(y | x) is linear in the unknown parameters that are estimated from the data. For this reason, polynomial regression is considered to be a special case of multiple linear regression.
y = 9450x + 25792
y = 16.393x2 + 9259.3x + 26215
y = -122.92x3 + 2099.4x2 - 718.71x + 38863
y = 4.9243x4 - 236.59x3 + 2979.9x2 - 3314.2x + 41165
y = 15.006x5 - 430.13x4 + 4409.7x3 - 19368x2 + 43652x + 8315
Code Starts Here
===============
import pandas as pd
Step 6 - Sampling
X_train, X_test, y_train, y_test = train_test_split(features,
labels,
test_size=0.33,
random_state=0)
Create the REgression Model
regressor = LinearRegression()
Create The Polynomial Features
poly_reg = PolynomialFeatures(degree=3)
Test the model
Calculate the Accuracy
for i in range(1,6):
poly_reg = PolynomialFeatures(degree=i)
print('Degree of Equation :', i)
All Playlist of this youtube channel
====================================
1. Data Preprocessing in Machine Learning
2. Confusion Matrix in Machine Learning, ML, AI
3. Anaconda, Python Installation, Spyder, Jupyter Notebook, PyCharm, Graphviz
4. Cross Validation, Sampling, train test split in Machine Learning
5. Drop and Delete Operations in Python Pandas
6. Matrices and Vectors with python
7. Detect Outliers in Machine Learning
8. TimeSeries preprocessing in Machine Learning
9. Handling Missing Values in Machine Learning
10. Dummy Encoding Encoding in Machine Learning
11. Data Visualisation with Python, Seaborn, Matplotlib
12. Feature Scaling in Machine Learning
13. Python 3 basics for Beginner
14. Statistics with Python
15. Sklearn Scikit Learn Machine Learning
16. Python Pandas Dataframe Operations
17. Linear Regression, Supervised Machine Learning
18 Interiew Questions on Machine Learning and Data Science
19. Jupyter Notebook Operations
Polynomial Linear Regression using Scikit Learn
In statistics, polynomial regression is a form of regression analysis in which the relationship between the independent variable x and the dependent variable y is modelled as an nth degree polynomial in x. Polynomial regression fits a nonlinear relationship between the value of x and the corresponding conditional mean of y, denoted E(y |x). Although polynomial regression fits a nonlinear model to the data, as a statistical estimation problem it is linear, in the sense that the regression function E(y | x) is linear in the unknown parameters that are estimated from the data. For this reason, polynomial regression is considered to be a special case of multiple linear regression.
y = 9450x + 25792
y = 16.393x2 + 9259.3x + 26215
y = -122.92x3 + 2099.4x2 - 718.71x + 38863
y = 4.9243x4 - 236.59x3 + 2979.9x2 - 3314.2x + 41165
y = 15.006x5 - 430.13x4 + 4409.7x3 - 19368x2 + 43652x + 8315
Code Starts Here
===============
import pandas as pd
Step 6 - Sampling
X_train, X_test, y_train, y_test = train_test_split(features,
labels,
test_size=0.33,
random_state=0)
Create the REgression Model
regressor = LinearRegression()
Create The Polynomial Features
poly_reg = PolynomialFeatures(degree=3)
Test the model
Calculate the Accuracy
for i in range(1,6):
poly_reg = PolynomialFeatures(degree=i)
print('Degree of Equation :', i)
All Playlist of this youtube channel
====================================
1. Data Preprocessing in Machine Learning
2. Confusion Matrix in Machine Learning, ML, AI
3. Anaconda, Python Installation, Spyder, Jupyter Notebook, PyCharm, Graphviz
4. Cross Validation, Sampling, train test split in Machine Learning
5. Drop and Delete Operations in Python Pandas
6. Matrices and Vectors with python
7. Detect Outliers in Machine Learning
8. TimeSeries preprocessing in Machine Learning
9. Handling Missing Values in Machine Learning
10. Dummy Encoding Encoding in Machine Learning
11. Data Visualisation with Python, Seaborn, Matplotlib
12. Feature Scaling in Machine Learning
13. Python 3 basics for Beginner
14. Statistics with Python
15. Sklearn Scikit Learn Machine Learning
16. Python Pandas Dataframe Operations
17. Linear Regression, Supervised Machine Learning
18 Interiew Questions on Machine Learning and Data Science
19. Jupyter Notebook Operations
Комментарии