filmov
tv
Uncertainty Modeling in AI | Lecture 12 (Part 2): Graph cut and alpha expansion
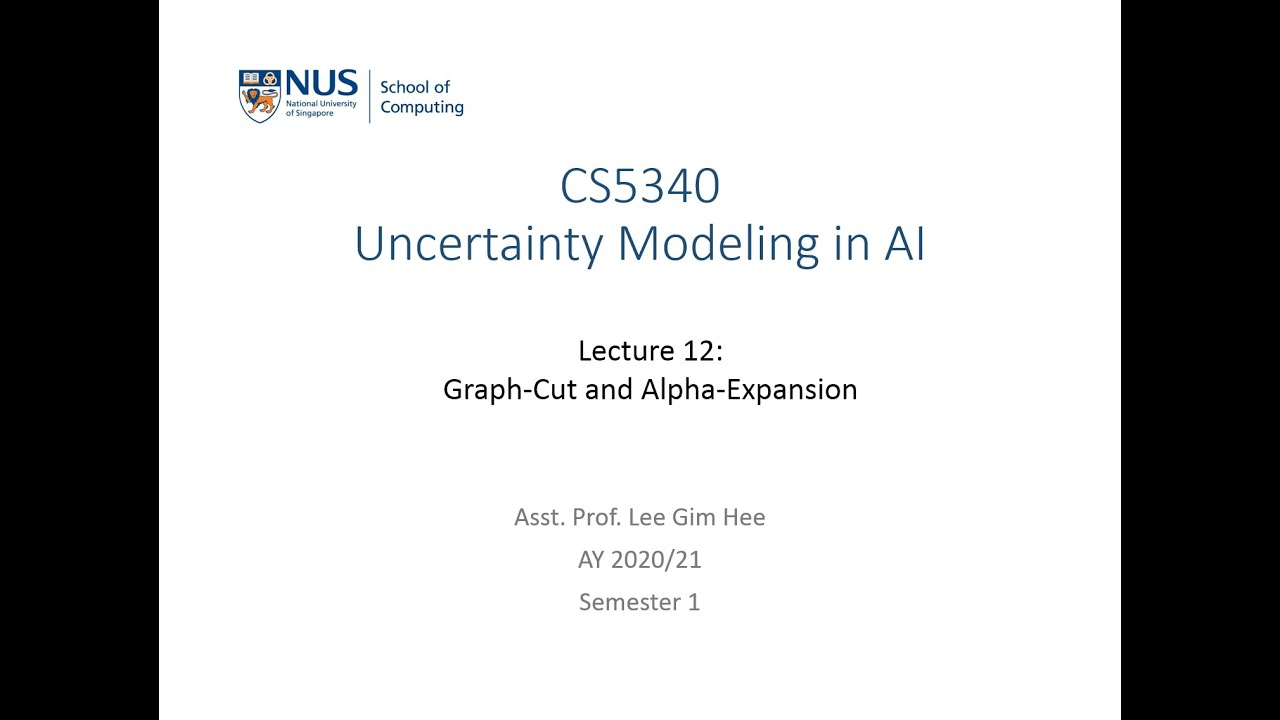
Показать описание
Here's the video lectures of CS5340 - Uncertainty Modeling in AI (Probabilistic Graphical Modeling) taught at the Department of Computer Science, National University of Singapore (NUS).
This is an introductory course on probabilistic graphical modeling which was recorded for online learning at NUS due to COVID-19. The topics covered include:
Lecture 1: Introduction to probabilistic reasoning
Lecture 2: Bayesian networks (Directed graphical models)
Lecture 3: Markov random fields (Undirected graphical models)
Lecture 4: Variable elimination and belief propagation
Lecture 5: Factor graph and the junction tree algorithm
Lecture 6: Parameter learning with complete data
Lecture 7: Mixture models and the EM algorithm
Lecture 8: Hidden Markov Models (HMM)
Lecture 9: Monte Carlo inference (Sampling)
Lecture 10: Variational inference
Lecture 11: Variational Auto Encoder and Mixture Density Networks
Lecture 12: Graph cut and alpha expansion
Disclaimer: This video lecture is provided freely for your reference. The lecturer and NUS are not responsible for anything expressed herein.
This is an introductory course on probabilistic graphical modeling which was recorded for online learning at NUS due to COVID-19. The topics covered include:
Lecture 1: Introduction to probabilistic reasoning
Lecture 2: Bayesian networks (Directed graphical models)
Lecture 3: Markov random fields (Undirected graphical models)
Lecture 4: Variable elimination and belief propagation
Lecture 5: Factor graph and the junction tree algorithm
Lecture 6: Parameter learning with complete data
Lecture 7: Mixture models and the EM algorithm
Lecture 8: Hidden Markov Models (HMM)
Lecture 9: Monte Carlo inference (Sampling)
Lecture 10: Variational inference
Lecture 11: Variational Auto Encoder and Mixture Density Networks
Lecture 12: Graph cut and alpha expansion
Disclaimer: This video lecture is provided freely for your reference. The lecturer and NUS are not responsible for anything expressed herein.
Uncertainty - Lecture 2 - CS50's Introduction to Artificial Intelligence with Python 2020
Uncertainty Modeling in AI | Lecture 1 (Part 1): Introduction to Probabilistic Reasoning
Nils Jansen - Neuro-Symbolic Artificial Intelligence: Intelligent Decisions Under Uncertainty
Florian Wilhelm: Are you sure about that?! Uncertainty Quantification in AI | PyData Berlin 2019
Uncertainty Modeling in AI | Lecture 10 (Part 1): Variational inference
Uncertainty (Aleatoric vs Epistemic) | Machine Learning
D1T8 Vasily Demyanov - Uncertainty in AI based reservoir modelling workflows
Uncertainty Modeling in AI | Lecture 2 (Part 1): Bayesian networks (Directed graphical models)
4th Applied Active Inference Symposium (Part 2, Nov 14, 2024) ~ Full Upload
Quantifying the Uncertainty in Model Predictions
Uncertainty Modeling in AI | Lecture 6 (Part 1): Parameter learning with complete data
[Artificial Intelligence] Show or Suppress? Managing Uncertainty in Model Explanations
Uncertainty in Statistical Modeling Explained Intuitively
Easy introduction to gaussian process regression (uncertainty models)
Uncertainty Modeling in AI | Lecture 9 (Part 1): Monte Carlo inference (Sampling)
Malte Tichy - Knowing what you don’t know matters- Uncertainty-aware model rating
AI Uncertainty: Boost Accuracy in Healthcare & Finance
Uncertainty in AI: Motivation
Uncertainty Quantification with Conformal Prediction: A Path to Reliable ML Models
Uncertainty Modeling in AI | Lecture 5 (Part 1): Factor graph and the junction tree algorithm
Reasoning under Uncertainty in Artificial Intelligence
Uncertainty Modeling in AI | Lecture 12 (Part 1): Graph cut and alpha expansion
Uncertainty Modeling in AI | Lecture 11 (Part 2): VAE and Mixture Density Networks
Uncertainty Modeling in AI | Lecture 7 (Part 1): Mixture models and the EM algorithm
Комментарии