filmov
tv
Tomer Michaeli - The implicit bias of SGD: A Dynamical stability analysis (Heb)
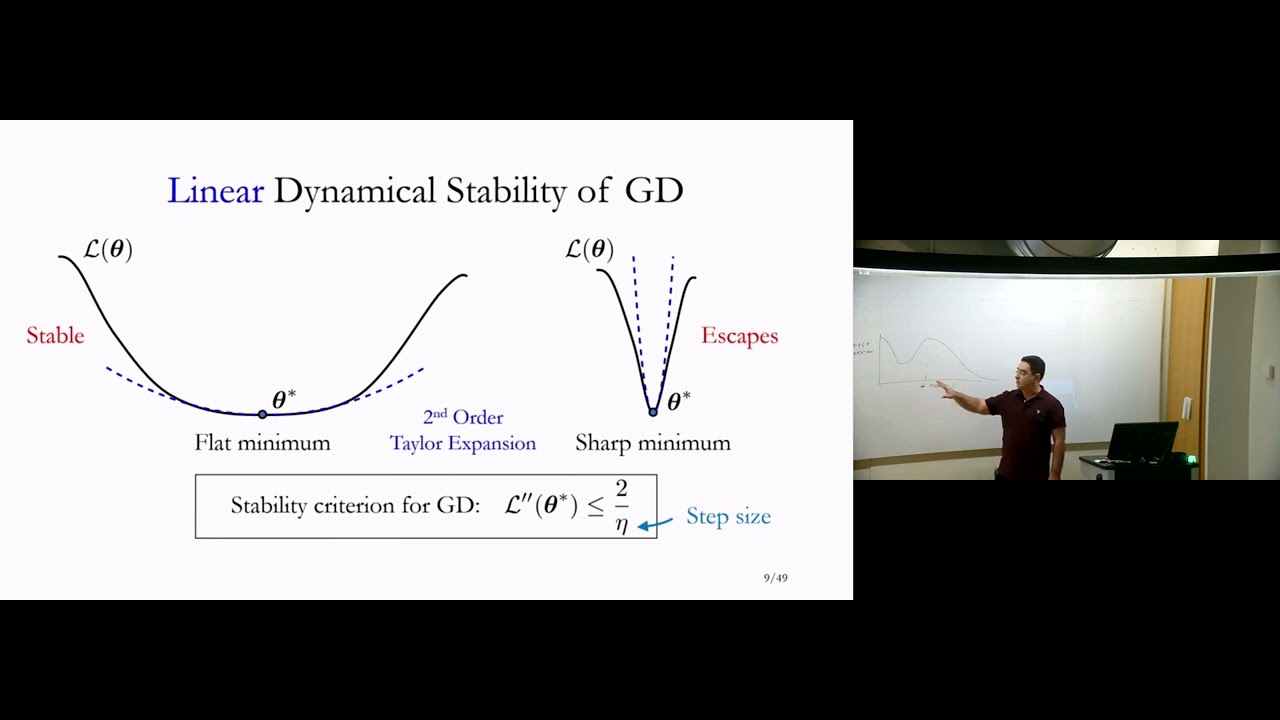
Показать описание
Presented on Thursday, October 31st, 2024, 10:30 AM, room C221
Speaker
Tomer Michaeli (Technion)
Title
The implicit bias of SGD: A Dynamical stability analysis
Abstract:
One of the puzzling phenomena in deep learning, is that neural networks tend to generalize well even when they are highly overparameterized. Recent works linked this behavior with the implicit biases of the optimization methods used to train networks (like SGD). Here, we analyze the implicit bias of SGD from the standpoint of the dynamical stability of the iterates at the vicinity of minima of the loss. Specifically, it is known that SGD can stably converge only to minima that are flat enough w.r.t. its step size. We prove that this property enforces the predictor function to become smoother as the step size increases, thus regularizing the solution. We also show that the family of solutions to which SGD can converge stably differs between depth-2 and depth-3 networks. Finally, we derive an explicit necessary and sufficient condition for the stability of SGD, improving upon the currently known necessary conditions. We demonstrate how our theoretical predictions align with practical experiments.
(Joint works with Rotem Mulayoff, Mor Shpigel Nacson, Greg Ongie, Daniel Soudry).
Bio:
Tomer Michaeli is an Associate Professor in the Faculty of Electrical and Computer Engineering at the Technion – Israel Institute of Technology. He completed his BSc and PhD degrees in this faculty in 2005 and 2012, respectively. From 2012 to 2015 he was a postdoctoral fellow in the CS and Applied Math Department at the Weizmann Institute of Science, and in 2015 he joined the Technion as a faculty member. His research lies in the fields of Computer Vision and Machine Learning. He is the recipient of several awards, among which are the Krill Prize for Excellence in Scientific Research by the Wolf foundation (2020), the Best Paper Award (Marr Prize) at ICCV 2019, and the Alon Fellowship for Outstanding Young Scientists (2017-2019).
Speaker
Tomer Michaeli (Technion)
Title
The implicit bias of SGD: A Dynamical stability analysis
Abstract:
One of the puzzling phenomena in deep learning, is that neural networks tend to generalize well even when they are highly overparameterized. Recent works linked this behavior with the implicit biases of the optimization methods used to train networks (like SGD). Here, we analyze the implicit bias of SGD from the standpoint of the dynamical stability of the iterates at the vicinity of minima of the loss. Specifically, it is known that SGD can stably converge only to minima that are flat enough w.r.t. its step size. We prove that this property enforces the predictor function to become smoother as the step size increases, thus regularizing the solution. We also show that the family of solutions to which SGD can converge stably differs between depth-2 and depth-3 networks. Finally, we derive an explicit necessary and sufficient condition for the stability of SGD, improving upon the currently known necessary conditions. We demonstrate how our theoretical predictions align with practical experiments.
(Joint works with Rotem Mulayoff, Mor Shpigel Nacson, Greg Ongie, Daniel Soudry).
Bio:
Tomer Michaeli is an Associate Professor in the Faculty of Electrical and Computer Engineering at the Technion – Israel Institute of Technology. He completed his BSc and PhD degrees in this faculty in 2005 and 2012, respectively. From 2012 to 2015 he was a postdoctoral fellow in the CS and Applied Math Department at the Weizmann Institute of Science, and in 2015 he joined the Technion as a faculty member. His research lies in the fields of Computer Vision and Machine Learning. He is the recipient of several awards, among which are the Krill Prize for Excellence in Scientific Research by the Wolf foundation (2020), the Best Paper Award (Marr Prize) at ICCV 2019, and the Alon Fellowship for Outstanding Young Scientists (2017-2019).