filmov
tv
The Green Revolution , SPATIAL AI in Agriculture
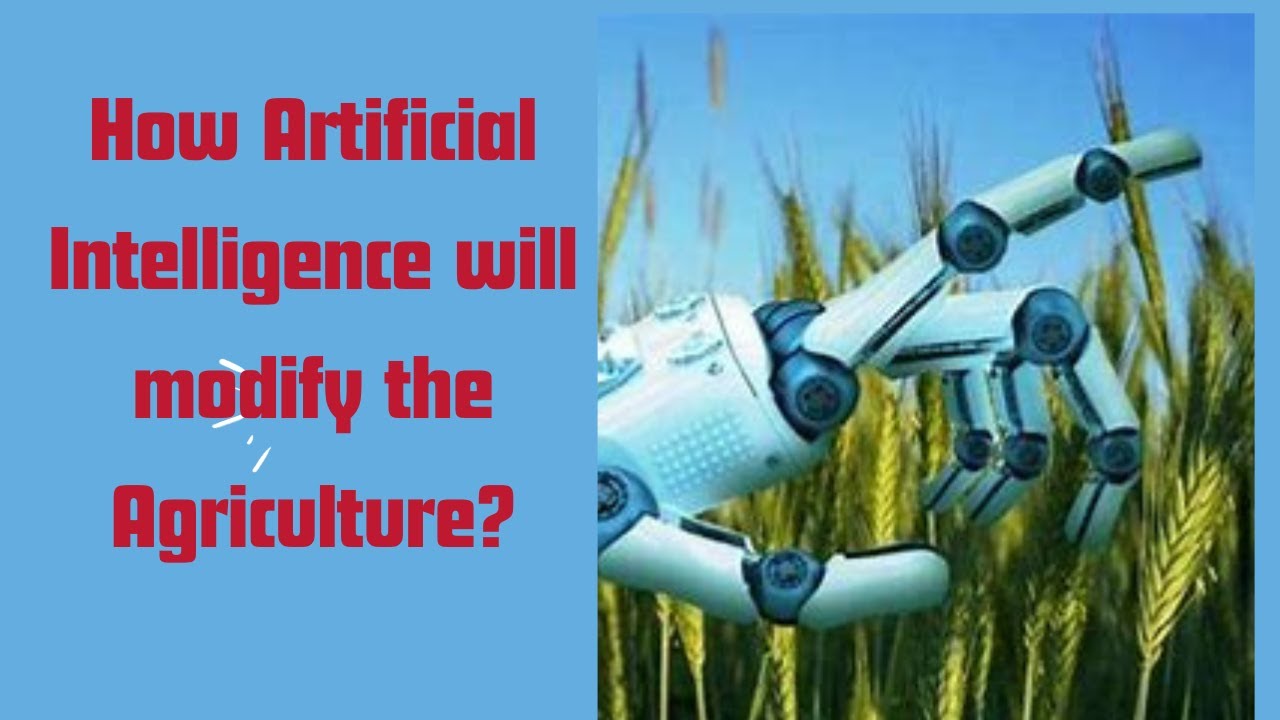
Показать описание
Spatial AI, or spatial artificial intelligence, can play a significant role in optimizing agricultural practices and improving overall efficiency. Here are some ways in which spatial AI can help in agriculture:
Precision Farming:
Satellite Imaging: Spatial AI utilizes satellite data to provide high-resolution imagery of agricultural fields. This helps farmers monitor crop health, identify areas with pest infestations, and optimize irrigation.
Drones: AI-powered drones equipped with cameras or sensors can capture detailed images of crops. These images can be analyzed to detect early signs of disease, nutrient deficiencies, or other issues, allowing for targeted interventions.
Crop Monitoring:
Remote Sensing: Spatial AI can analyze data from various remote sensing technologies to monitor crop growth, assess vegetation health, and predict yield. This information helps farmers make informed decisions about fertilization, irrigation, and harvesting.
Predictive Analytics:
Weather Forecasting: Integrating spatial AI with weather data allows farmers to make better decisions based on upcoming weather conditions. This helps in planning activities like planting, harvesting, and irrigation, minimizing risks associated with adverse weather.
Soil Analysis:
GIS (Geographic Information System): Combining spatial AI with GIS enables detailed soil analysis. Farmers can understand soil composition, moisture levels, and nutrient content, allowing for precise and targeted application of fertilizers and other inputs.
Automated Machinery:
Autonomous Vehicles: Spatial AI can be used to enable autonomous machinery for tasks like planting, weeding, and harvesting. These machines can navigate fields with precision, reducing labor costs and optimizing resource use.
Supply Chain Optimization:
Logistics Planning: Spatial AI can assist in optimizing the supply chain by analyzing transportation routes, predicting demand, and identifying efficient distribution strategies. This helps in reducing waste and ensuring timely delivery of produce.
Crop Modeling:
Machine Learning Models: Spatial AI can leverage machine learning algorithms to create models that predict crop growth patterns, disease outbreaks, and yield. These models improve over time as they are fed more data, leading to increasingly accurate predictions.
Resource Management:
Water Management: Spatial AI can aid in optimizing water usage by providing insights into soil moisture levels and predicting irrigation needs. This is especially valuable in regions facing water scarcity.
In summary, spatial AI enhances decision-making in agriculture by providing real-time insights, optimizing resource utilization, and improving overall efficiency. This technology has the potential to revolutionize traditional farming practices, making them more sustainable and productive.
Precision Farming:
Satellite Imaging: Spatial AI utilizes satellite data to provide high-resolution imagery of agricultural fields. This helps farmers monitor crop health, identify areas with pest infestations, and optimize irrigation.
Drones: AI-powered drones equipped with cameras or sensors can capture detailed images of crops. These images can be analyzed to detect early signs of disease, nutrient deficiencies, or other issues, allowing for targeted interventions.
Crop Monitoring:
Remote Sensing: Spatial AI can analyze data from various remote sensing technologies to monitor crop growth, assess vegetation health, and predict yield. This information helps farmers make informed decisions about fertilization, irrigation, and harvesting.
Predictive Analytics:
Weather Forecasting: Integrating spatial AI with weather data allows farmers to make better decisions based on upcoming weather conditions. This helps in planning activities like planting, harvesting, and irrigation, minimizing risks associated with adverse weather.
Soil Analysis:
GIS (Geographic Information System): Combining spatial AI with GIS enables detailed soil analysis. Farmers can understand soil composition, moisture levels, and nutrient content, allowing for precise and targeted application of fertilizers and other inputs.
Automated Machinery:
Autonomous Vehicles: Spatial AI can be used to enable autonomous machinery for tasks like planting, weeding, and harvesting. These machines can navigate fields with precision, reducing labor costs and optimizing resource use.
Supply Chain Optimization:
Logistics Planning: Spatial AI can assist in optimizing the supply chain by analyzing transportation routes, predicting demand, and identifying efficient distribution strategies. This helps in reducing waste and ensuring timely delivery of produce.
Crop Modeling:
Machine Learning Models: Spatial AI can leverage machine learning algorithms to create models that predict crop growth patterns, disease outbreaks, and yield. These models improve over time as they are fed more data, leading to increasingly accurate predictions.
Resource Management:
Water Management: Spatial AI can aid in optimizing water usage by providing insights into soil moisture levels and predicting irrigation needs. This is especially valuable in regions facing water scarcity.
In summary, spatial AI enhances decision-making in agriculture by providing real-time insights, optimizing resource utilization, and improving overall efficiency. This technology has the potential to revolutionize traditional farming practices, making them more sustainable and productive.