filmov
tv
Introduction to machine learning, Part 5: The proper-orthogonal decomposition (POD)
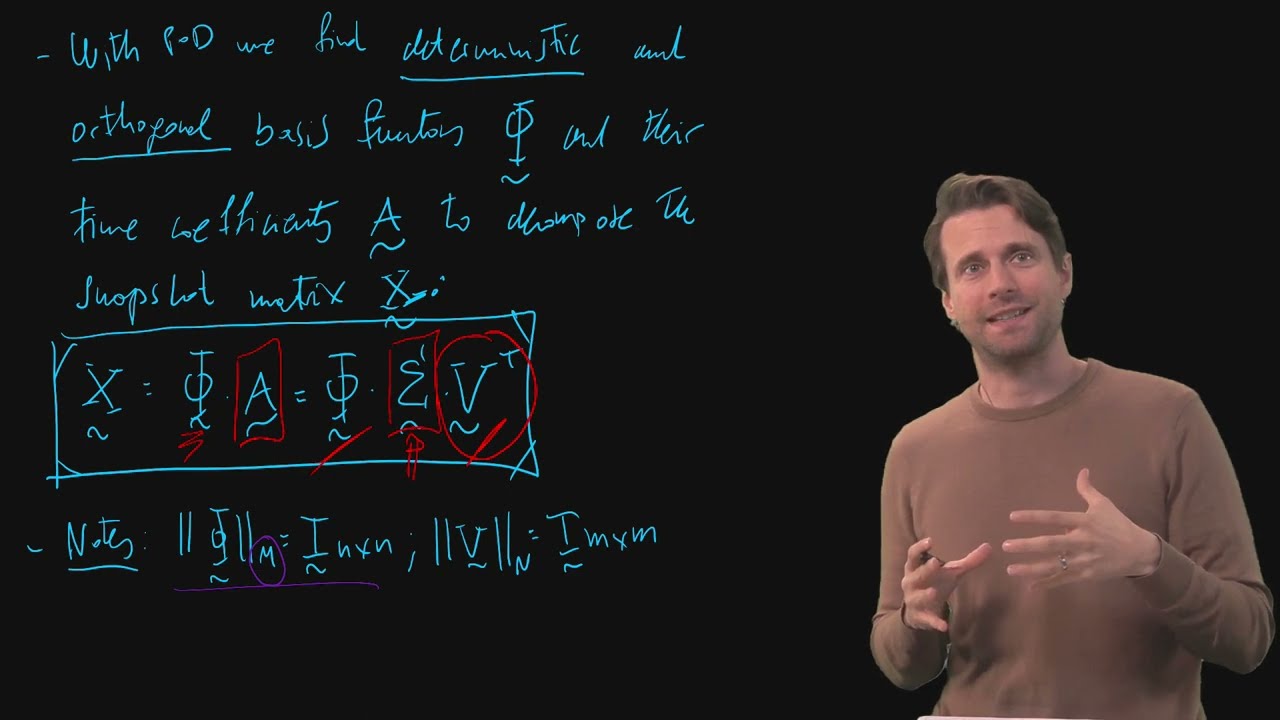
Показать описание
To conclude this first introduction series to ML, we derive and describe in detail a widely used method for analysis of high-dimensional fluid-flow systems: the proper-orthogonal decomposition (POD).
I also acknowledge Scott Dawson for his input on this material.
I also acknowledge Scott Dawson for his input on this material.
Machine Learning | What Is Machine Learning? | Introduction To Machine Learning | 2024 | Simplilearn
Intro to Machine Learning (ML Zero to Hero - Part 1)
INTRODUCTION TO MACHINE LEARNING: PART ONE
Machine Learning Fundamentals | Introduction to Machine Learning, Part 1
11. Introduction to Machine Learning
Introduction to Machine Learning-Part-1Theory
Machine Learning for Everybody – Full Course
Introduction to Machine Learning
Mastering AI Communication:😎Top Prompting Tips! #shorts #aigenerated
A Gentle Introduction to Machine Learning
AI vs Machine Learning
What is Machine Learning?
But what is a neural network? | Chapter 1, Deep learning
Introduction to Machine Learning
Machine Learning Explained in 100 Seconds
All Machine Learning Models Explained in 5 Minutes | Types of ML Models Basics
Getting Started with Machine Learning | Introduction to Machine Learning, Part 4
Introduction to ML and AI - MFML Part 1
What is Machine Learning? | Machine Learning Basics | Machine Learning Tutorial | Edureka
Machine Learning In 5 Minutes | Machine Learning Introduction |What Is Machine Learning |Simplilearn
INTRODUCTION TO MACHINE LEARNING: PART TWO
Andrew Ng's Secret to Mastering Machine Learning - Part 1 #shorts
Python Machine Learning Tutorial (Data Science)
Supervised vs Unsupervised vs Reinforcement Learning | Machine Learning Tutorial | Simplilearn
Комментарии