filmov
tv
Pydantic Tutorial • Solving Python's Biggest Problem
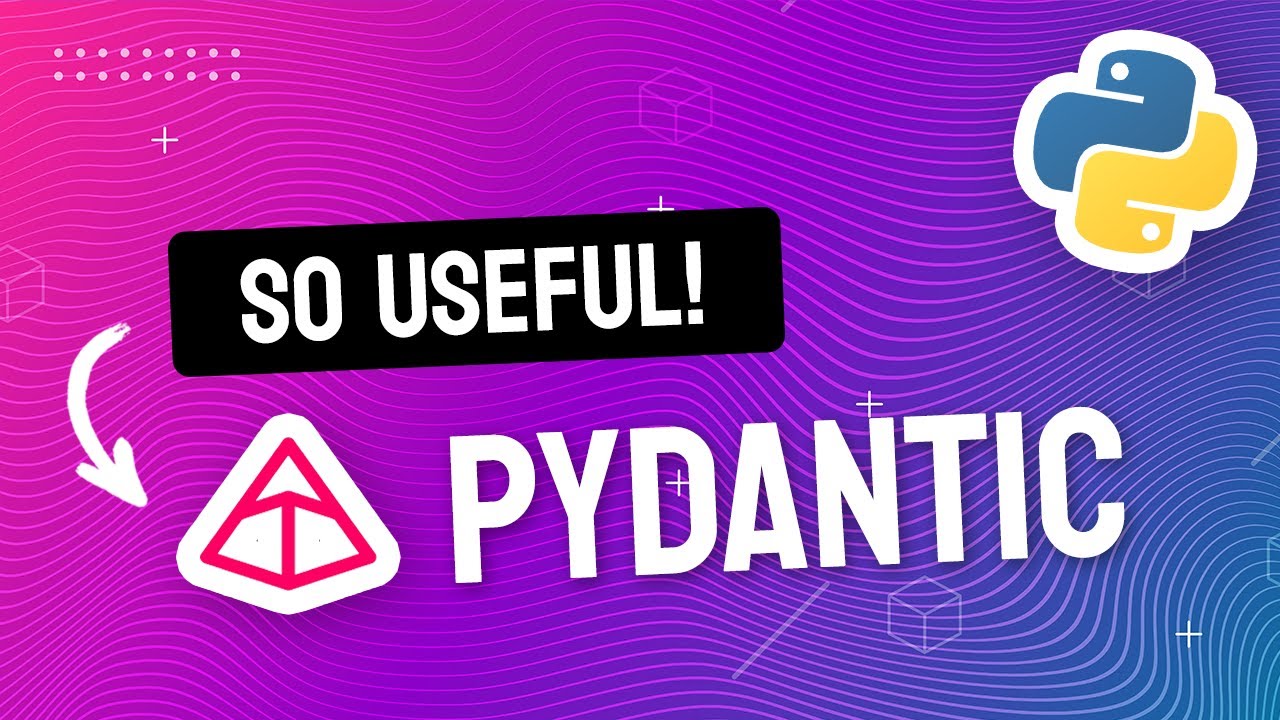
Показать описание
Learn how to use Pydantic in this short tutorial!
Pydantic is the most widely used data validation library for Python. It lets you structure your data, gives you type-hints and auto-complete in your IDE, and helps to serialize to and from JSON. Learn how to use it in just 10 minutes!
👉 Links
📚 Chapters
00:00 Python's Dynamic Typing Problem
02:11 How To Use Pydantic
05:04 Validating Data with Pydantic
06:36 Custom Field Validation
07:58 JSON Serialization
08:49 Pydantic vs Dataclasses
#pixegami #python
Pydantic is the most widely used data validation library for Python. It lets you structure your data, gives you type-hints and auto-complete in your IDE, and helps to serialize to and from JSON. Learn how to use it in just 10 minutes!
👉 Links
📚 Chapters
00:00 Python's Dynamic Typing Problem
02:11 How To Use Pydantic
05:04 Validating Data with Pydantic
06:36 Custom Field Validation
07:58 JSON Serialization
08:49 Pydantic vs Dataclasses
#pixegami #python
Pydantic tutorial • solving python's biggest problem
Do We Still Need Dataclasses? // PYDANTIC Tutorial
Attrs, Pydantic, or Python Data Classes?
Streamlining Data Preparation with Pydantic: A 25-Minute Guide | Python Data Science Day
Pydantic Introduction - Models, Fields, Constrained Types, Validator Functions and Model Exports
Which Python @dataclass is best? Feat. Pydantic, NamedTuple, attrs...
Intro to Pydantic V1
Does Pydantic Replace Dataclasses in Python?
Pydantic: Migrating from V1 to V2
Fastapi Python Complete Tutorial|Request body and pydantic model In Fastapi |Tutorial:9
327: Untangling XML with Pydantic
Build a Full-Stack ML Application With Pydantic And Prefect
FastUI & Pydantic - Build Interactive UIs with Declarative Python code
This Is Why Python Data Classes Are Awesome
Making a POST Request with FastAPI and Pydantic | Python Tutorial
Replace your YAML configs with Pydantic
FastAPI Creating Models using Pydantic - Data Validation module
Making a GET Request with FastAPI and Pydantic | Python Tutorial
From Pydantic V1 to V2 - Hemangi Subodh Karchalkar
Easy Data Validation For Your Python Projects With Pydantic
How To Write Unit Tests For Existing Python Code // Part 1 of 2
343: So Much Pydantic! - Python Bytes
Python 3.12 Generic Types Explained
Pydantic v2, the Plan - Talk Python to Me Ep. 376
Комментарии