filmov
tv
DALL-E 3 is better at following Text Prompts! Here is why. — DALL-E 3 explained
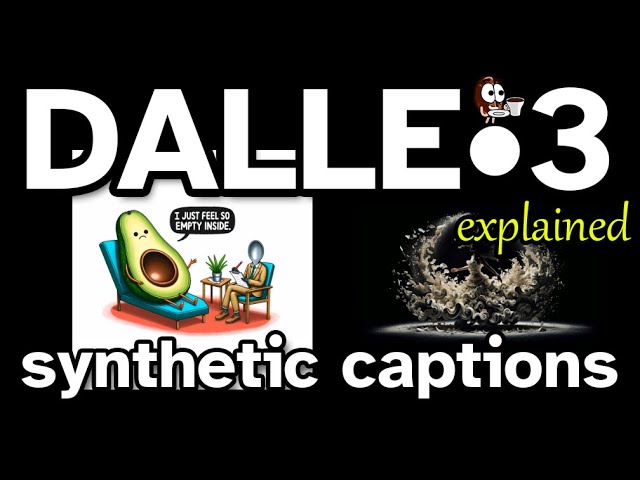
Показать описание
Synthetic captions help DALL-E 3 follow text prompts better than DALL-E 2. We explain how OpenAI innovates the training of diffusion models with better image captions.
Thanks to our Patrons who support us in Tier 2, 3, 4: 🙏
Dres. Trost GbR, Siltax, Vignesh Valliappan, Mutual Information, Kshitij
Outline:
00:00 DALLE-3
00:41 Gradient (Sponsor)
01:50 Timeline of image generation
03:34 Recaptioning with synthetic captions
04:36 Creating the synthetic captions
05:19 How well does it work?
▀▀▀▀▀▀▀▀▀▀▀▀▀▀▀▀▀▀▀▀▀▀▀▀▀▀
🔥 Optionally, pay us a coffee to help with our Coffee Bean production! ☕
▀▀▀▀▀▀▀▀▀▀▀▀▀▀▀▀▀▀▀▀▀▀▀▀▀▀
🔗 Links:
#AICoffeeBreak #MsCoffeeBean #MachineLearning #AI #research
Music 🎵 : 368 - Dyalla
Video editing: Nils Trost
Thanks to our Patrons who support us in Tier 2, 3, 4: 🙏
Dres. Trost GbR, Siltax, Vignesh Valliappan, Mutual Information, Kshitij
Outline:
00:00 DALLE-3
00:41 Gradient (Sponsor)
01:50 Timeline of image generation
03:34 Recaptioning with synthetic captions
04:36 Creating the synthetic captions
05:19 How well does it work?
▀▀▀▀▀▀▀▀▀▀▀▀▀▀▀▀▀▀▀▀▀▀▀▀▀▀
🔥 Optionally, pay us a coffee to help with our Coffee Bean production! ☕
▀▀▀▀▀▀▀▀▀▀▀▀▀▀▀▀▀▀▀▀▀▀▀▀▀▀
🔗 Links:
#AICoffeeBreak #MsCoffeeBean #MachineLearning #AI #research
Music 🎵 : 368 - Dyalla
Video editing: Nils Trost
DALL-E 3: KI-Bilder kostenlos erstellen! Besser als Midjourney?
How to Use DALL.E 3 - Top Tips for Best Results
🖼️ DALL-E 3 + ChatGPT im Test 👉🏻 Bilder in ChatGPT erstellen
Midjourney V6 VS DALL•E 3: Prompt Battle & Full Review
DALL-E 3 in ChatGPT | Tipps & Tricks die du kennen solltest
DALLE-3 Just Killed Midjourney & Every Text-To-image Tool 😱
OpenAI’s DALL-E 3-Like AI For Free, Forever!
Dall-E - How To PROMPT Like A PRO (In 3 Minutes!!!)
Prezenty na święta TOP 3
Konsistente Charaktere mit KI? So machst du es mit DALL·E 3 & Leonardo AI!
DALL-E 2 Tutorial für Anfänger | Bilder erstellen & bearbeiten mit Künstlicher Intelligenz
DALL-E 3: Der ultimative Game Changer in der Bilder-KI! ChatGPT Meisterstück
DALL-E 3 + ChatGPT ist der HAMMER! 🔥 Alle Funktionen + Tests [deutsch]
Which is better? Midjourney v6 vs. DALL-E 3 vs. Stable Diffusion XL
RIESENUPGRADE FÜR CHATGPT – Mit Dall-E 3 kann ChatGPT Bilder generieren
DALL-E 3 kostenlos Bilder mit KI erstellen - Open AI Text Generator in Microsoft Bing [deutsch]
DALLE 3 Is Now FREE And Just Killed Midjourney😱
What is Dall-E? (in about a minute)
DALL·E 3 jetzt kostenlos nutzen | KI Bilder wie nie zuvor
Midjourney vs DALL E 3 Prompt Battle Best AI Image Generator
You can now access DALL-E 3 right inside of ChatGPT
Use DALL E 3 for free with this! 🚀
Consistent Characters - FREE - NO Midjourney AI - NO Dalle-3 - NO Leonardo AI
Ranking The Best AI Image Generation Tools
Комментарии