filmov
tv
How you can create pictures like Van Gogh. Neural Style Transfer Introduced. Machine Learning Terms.
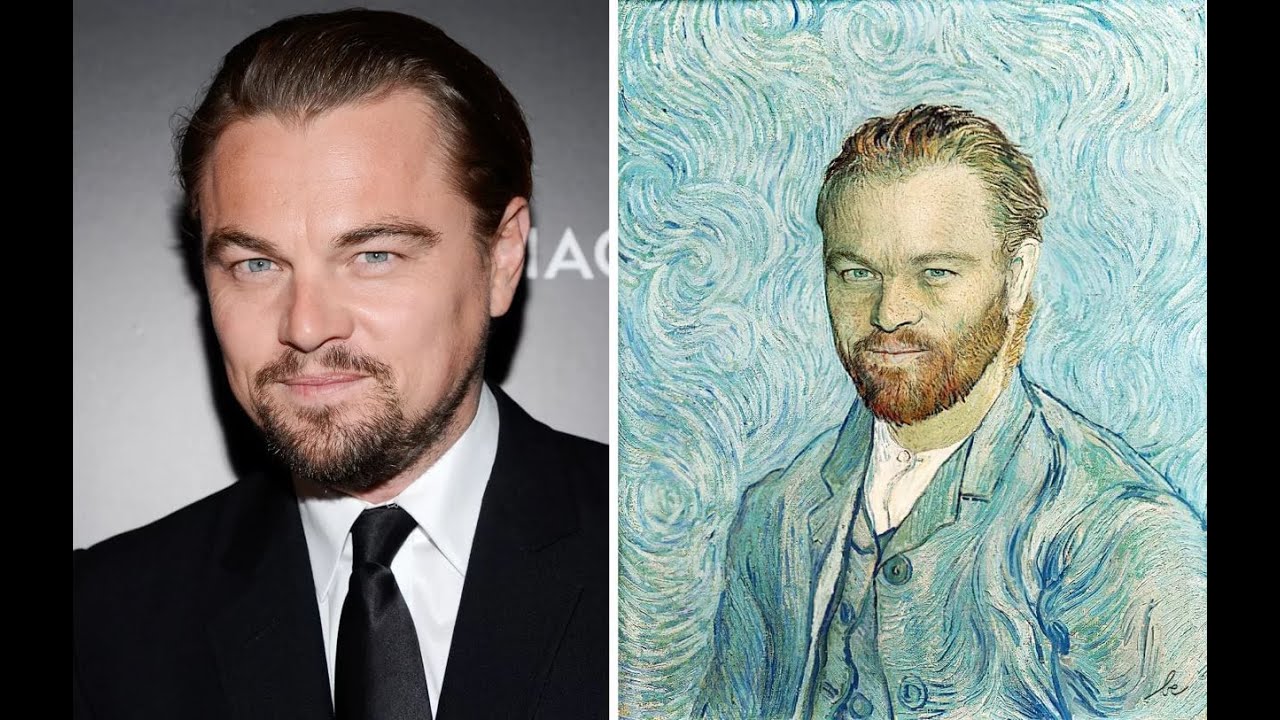
Показать описание
Neural Style Transfer (NST) refers to a class of software algorithms that manipulate digital images, or videos, in order to adopt the appearance or visual style of another image. NST algorithms are characterized by their use of deep neural networks for the sake of image transformation. Common uses for NST are the creation of artificial artwork from photographs, for example by transferring the appearance of famous paintings to user-supplied photographs. Several notable mobile apps use NST techniques for this purpose, including DeepArt and Prisma. This method has been used by artists and designers around the globe to develop new artwork based on existent style(s).
For example, we can take an image of a suburban neighborhood, and another image of Van Gogh's Starry Night. Then we use the Neural Style Transfer to transfer the style of the Starry Night onto the image of the neighborhood.
Neural Style Transfer has also been used to create better training data for medical images. For more details check out: SinGAN-Seg: How it solves the problem of small datasets and private data in Medical Machine Learning.
This is part of my series Machine Learning Terms. Here I share various #ML and #AI terms in less than a minute to help you learn about more ideas and concepts. With these concise videos, you will know the basics of diverse machine learning concepts.
Paper Details on SinGAN-Seg.
SinGAN-Seg: Synthetic Training Data Generation for Medical Image Segmentation
Vajira Thambawita, Pegah Salehi, Sajad Amouei Sheshkal, Steven A. Hicks, Hugo L.Hammer, Sravanthi Parasa, Thomas de Lange, Pål Halvorsen, Michael A. Riegler
Processing medical data to find abnormalities is a time-consuming and costly task, requiring tremendous efforts from medical experts. Therefore, Ai has become a popular tool for the automatic processing of medical data, acting as a supportive tool for doctors. AI tools highly depend on data for training the models. However, there are several constraints to access to large amounts of medical data to train machine learning algorithms in the medical domain, e.g., due to privacy concerns and the costly, time-consuming medical data annotation process. To address this, in this paper we present a novel synthetic data generation pipeline called SinGAN-Seg to produce synthetic medical data with the corresponding annotated ground truth masks. We show that these synthetic data generation pipelines can be used as an alternative to bypass privacy concerns and as an alternative way to produce artificial segmentation datasets with corresponding ground truth masks to avoid the tedious medical data annotation process. As a proof of concept, we used an open polyp segmentation dataset. By training UNet++ using both the real polyp segmentation dataset and the corresponding synthetic dataset generated from the SinGAN-Seg pipeline, we show that the synthetic data can achieve a very close performance to the real data when the real segmentation datasets are large enough. In addition, we show that synthetic data generated from the SinGAN-Seg pipeline improving the performance of segmentation algorithms when the training dataset is very small. Since our SinGAN-Seg pipeline is applicable for any medical dataset, this pipeline can be used with any other segmentation datasets.
Subjects: Image and Video Processing (eess.IV); Computer Vision and Pattern Recognition (cs.CV); Machine Learning (cs.LG)
Cite as: arXiv:2107.00471 [eess.IV]
(or arXiv:2107.00471v1 [eess.IV] for this version)
Reach out to me:
#MachineLearning #Cancer #Medicine #NoisyInput #Detection #ImageClassification #Google #Facebook #Devansh #MachineLearningMadeSimple #DeepLearning #CNN #Convolution #ANNs #NeuralNetworks #AI #ArtificialIntelligence #SoftwareDevelopment #SigmoidFunction #Math #Art #VanGogh
For example, we can take an image of a suburban neighborhood, and another image of Van Gogh's Starry Night. Then we use the Neural Style Transfer to transfer the style of the Starry Night onto the image of the neighborhood.
Neural Style Transfer has also been used to create better training data for medical images. For more details check out: SinGAN-Seg: How it solves the problem of small datasets and private data in Medical Machine Learning.
This is part of my series Machine Learning Terms. Here I share various #ML and #AI terms in less than a minute to help you learn about more ideas and concepts. With these concise videos, you will know the basics of diverse machine learning concepts.
Paper Details on SinGAN-Seg.
SinGAN-Seg: Synthetic Training Data Generation for Medical Image Segmentation
Vajira Thambawita, Pegah Salehi, Sajad Amouei Sheshkal, Steven A. Hicks, Hugo L.Hammer, Sravanthi Parasa, Thomas de Lange, Pål Halvorsen, Michael A. Riegler
Processing medical data to find abnormalities is a time-consuming and costly task, requiring tremendous efforts from medical experts. Therefore, Ai has become a popular tool for the automatic processing of medical data, acting as a supportive tool for doctors. AI tools highly depend on data for training the models. However, there are several constraints to access to large amounts of medical data to train machine learning algorithms in the medical domain, e.g., due to privacy concerns and the costly, time-consuming medical data annotation process. To address this, in this paper we present a novel synthetic data generation pipeline called SinGAN-Seg to produce synthetic medical data with the corresponding annotated ground truth masks. We show that these synthetic data generation pipelines can be used as an alternative to bypass privacy concerns and as an alternative way to produce artificial segmentation datasets with corresponding ground truth masks to avoid the tedious medical data annotation process. As a proof of concept, we used an open polyp segmentation dataset. By training UNet++ using both the real polyp segmentation dataset and the corresponding synthetic dataset generated from the SinGAN-Seg pipeline, we show that the synthetic data can achieve a very close performance to the real data when the real segmentation datasets are large enough. In addition, we show that synthetic data generated from the SinGAN-Seg pipeline improving the performance of segmentation algorithms when the training dataset is very small. Since our SinGAN-Seg pipeline is applicable for any medical dataset, this pipeline can be used with any other segmentation datasets.
Subjects: Image and Video Processing (eess.IV); Computer Vision and Pattern Recognition (cs.CV); Machine Learning (cs.LG)
Cite as: arXiv:2107.00471 [eess.IV]
(or arXiv:2107.00471v1 [eess.IV] for this version)
Reach out to me:
#MachineLearning #Cancer #Medicine #NoisyInput #Detection #ImageClassification #Google #Facebook #Devansh #MachineLearningMadeSimple #DeepLearning #CNN #Convolution #ANNs #NeuralNetworks #AI #ArtificialIntelligence #SoftwareDevelopment #SigmoidFunction #Math #Art #VanGogh