filmov
tv
Why You Need More Than Airflow // Ketan Umare // Coffee Sessions #109
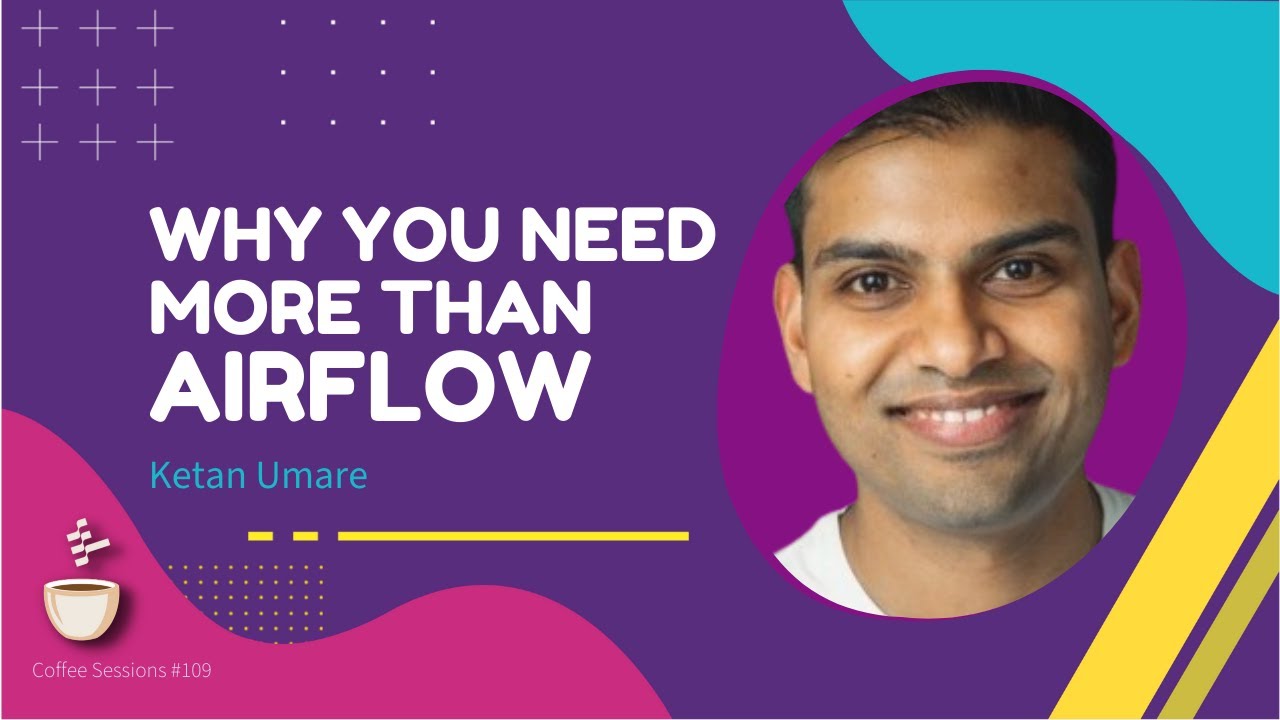
Показать описание
// Abstract
Airflow is a beloved tool by data engineers and Machine Learning Engineers alike. But when doing ML what are the shortcomings and why is an orchestration tool like that not always the best developer experience? In this episode, we break down what some key drivers are for using an ML-specific orchestration tool.
// Bio
// MLOps Jobs board
MLOps Swag/Merch
// Related Links
--------------- ✌️Connect With Us ✌️ -------------
Follow us on Twitter: @mlopscommunity
Timestamps:
[00:00] Introduction to Ketan Umare
[01:08] Takeaways
[04:03] Changes with Ketan after 2 years
[10:06] Diametrically opposed software and data infrastructure
[13:06] Airflow shortcomings
[21:14] Core abstractions
[24:56] Machine Learning specific packages
[28:25] Core target users
[30:50] Airflow foundational level
[33:46] Better visualization of data science pipelines
[36:39] Flyte for RL Deployment
[38:36] Role of signals in the system
[40:59] Everything in Flyte is run through an API
[42:20] Manual intervention in the system
[45:11] Union vs Flyte
[50:16] Security precautions
[51:56] Role of Union cloud
[56:20] Distinctiveness of Flyte pipelines
[01:01:11] Ketan in his current company
[01:02:25] Learning from Airflow
[01:04:58] Lightning round with Ketan
[01:09:59] Wrap up