filmov
tv
Seminar | Tim Duignan | Recursive neural network potentials solve the connecting scales problem
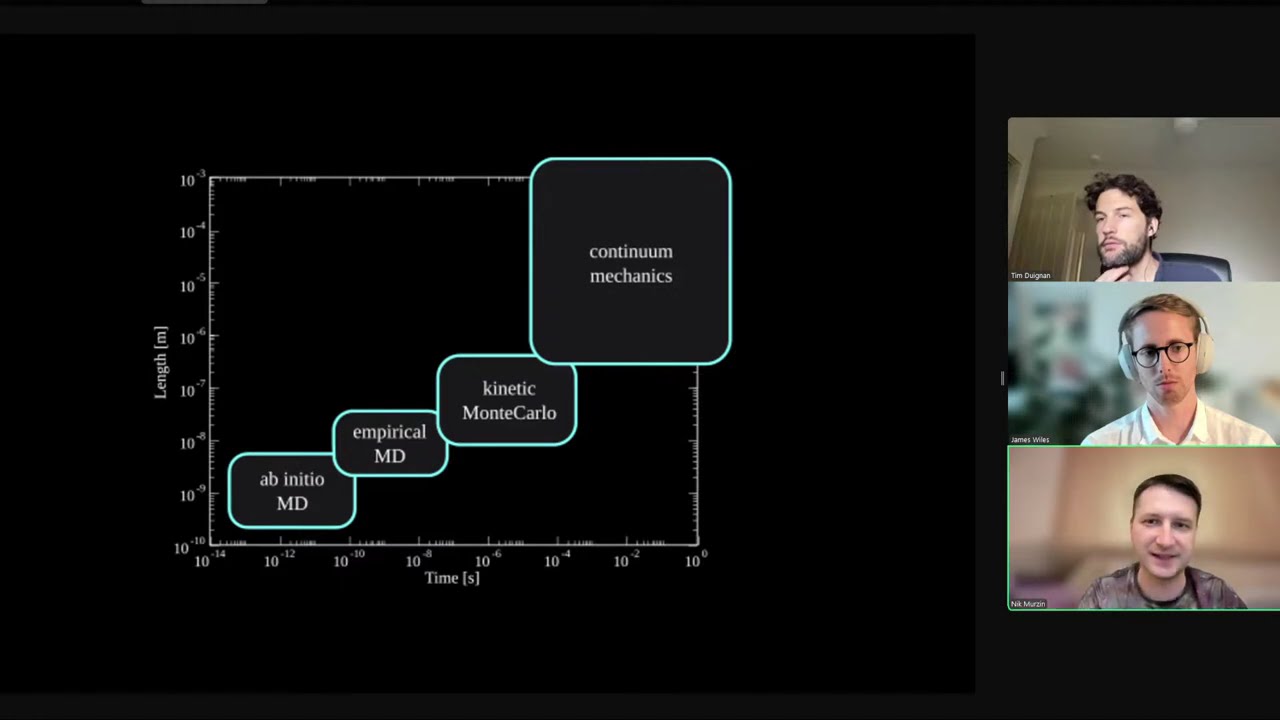
Показать описание
"In the next half-century, physical chemistry will likely undergo a profound transformation, driven predominantly by the combination of recent advances in quantum chemistry and machine learning (ML). Specifically, equivariant neural network potentials (NNPs) are a breakthrough new tool that are already enabling us to simulate systems at the molecular scale with unprecedented accuracy and speed, relying on nothing but fundamental physical laws. The continued development of this approach will realize Paul Dirac’s 80-year-old vision of using quantum mechanics to unify physics with chemistry and providing invaluable tools for understanding materials science, biology, earth sciences, and beyond. The era of highly accurate and efficient first-principles molecular simulations will provide a wealth of training data that can be used to build automated computational methodologies, using tools such as diffusion models, for the design and optimization of systems at the molecular scale. Large language models (LLMs) will also evolve into increasingly indispensable tools for literature review, coding, idea generation, and scientific writing."
Participants:
Tim Duignan, Dugan Hammock, Nikolay Murzin, Carlos Zapata-Carratalá, Richard Assar, James Wiles.
Materials:
References:
- Scalable molecular simulation of electrolyte solutions with quantum chemical accuracy
Keywords:
molecular simulation, molecular dynamics, machine learning, artificial intelligence, coarse-graining, large language models, diffusion models
Participants:
Tim Duignan, Dugan Hammock, Nikolay Murzin, Carlos Zapata-Carratalá, Richard Assar, James Wiles.
Materials:
References:
- Scalable molecular simulation of electrolyte solutions with quantum chemical accuracy
Keywords:
molecular simulation, molecular dynamics, machine learning, artificial intelligence, coarse-graining, large language models, diffusion models