filmov
tv
Elections, Public Opinion & Data Science - nyhackr x NYAAPOR October Meetup
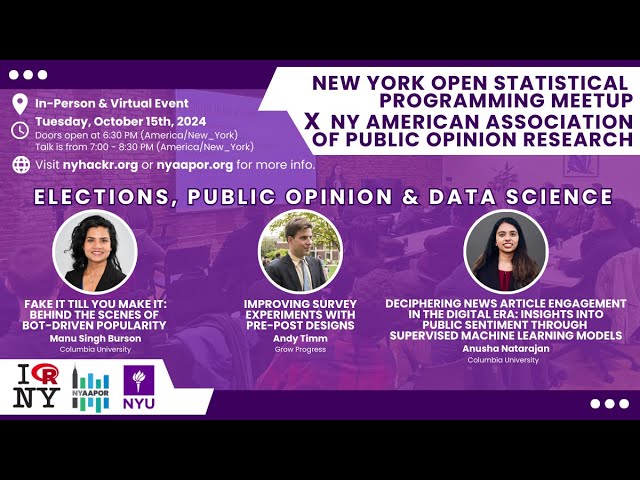
Показать описание
We teamed up with NYAAPOR to give you Elections, Public Opinion & Data Science - 3 Lightning Talks
Speaker - Manu Singh Burson (Computational Social Scientist and Doctoral Candidate in Political Science at Columbia University)
Talk - Fake it Till You Make it: Behind the Scenes of Bot-Driven Popularity
Talk Description - My paper examines how fake social media accounts boost politicians' online popularity and this phenomenon's subsequent spillover on traditional news coverage. Using the 'Botometer' algorithm, I assessed the proportion of bot accounts engaging with tweets from 382 U.S. Congress members on Twitter. A policy change to Twitter's API infrastructure in November 2022 was an exogenous shock to the platform that significantly hampered bot functionality. My first-stage analysis demonstrated that this policy change only affected high-bot-engagement politicians, who saw a substantial decline in followers after November 2022. Placebo comparisons show this decline was not observed in comparable data from Facebook 'likes' or Instagram followers. My second-stage analysis found that after the policy change, high-bot-engagement politicians also saw a decline in coverage in digital news articles and TV news from December 2022 to February 2024.
_______
Speaker - Andy Timm (Senior Data Scientist at Grow Progress)
Talk - Improving Survey Experiments with Pre-Post Designs
Talk Description - Grow Progress has run well over a thousand survey experiments on behalf of our politics and advocacy clients in the 2024 cycle. This type of pre-testing creative before a broader rollout is increasingly a best practice in campaign work, with most major campaigns, party bodies, and large PACs extensively testing their ads. These tests can often be made significantly more precise via the use of a pre-post design, but not all tests benefit overall from this setup. In this talk, I'll share some internal research clarifying which experiments tend to benefit most from these designs, including a simulation study, and results from experiments that are well-powered to detect the bias of pre-post vs. a more typical survey experiment.
_______
Speaker - Anusha Natarajan (Graduate Student at Columbia University)
Talk - Deciphering News Article Engagement in the Digital Era: Insights into Public Sentiment through Supervised Machine Learning Models
Talk Description - 2020 was marked with a series of unprecedented events, such as the pandemic, the murder of George Floyd, and the crucial 2020 U.S. presidential election. In a survey conducted by the Pew Research Center in 2020, a little over half of the respondents (53%) claim that they got their news from social media and digital platforms. Thus, an increasing number of individuals actively participated in discussions on various platforms, including commenting on media platforms or posting on social media like Twitter. As discussions around these events proliferated across social media and news outlets, understanding the factors driving the popularity of articles became paramount. Articles were collected from The New York Times between 01/01/2020 to 12/31/2020 to understand what features of user engagement and the characteristics of the article itself have with popularity. A total of 16,787 articles were included in our analysis, with information on the article's section, headline, abstract, keyword, word count, publication date, number of comments, sentiment, and popularity recorded. Supervised machine learning models, including linear, ridge, lasso, random forest, and gradient boosting regressions, were employed to understand the data through utilizing R. Based on feature selection in using the random forest, factors like publication date (0.324), section (0.305), and word count (0.371) significantly impact article engagement, while sentiment had no influence over the popularity of an article. Using those features, top sections and keywords were identified from popular articles, while exploring temporal trends to gauge discourse intensity during specific periods. Analysis of top sections with the highest comment counts revealed keywords centered around major events like COVID-19, the 2020 election, and the killing of George Floyd, with engagement peaking during the summer of 2020.