filmov
tv
PathPartner's Praveen G.B. Explores CNN Architectures for Face Recognition (Preview)
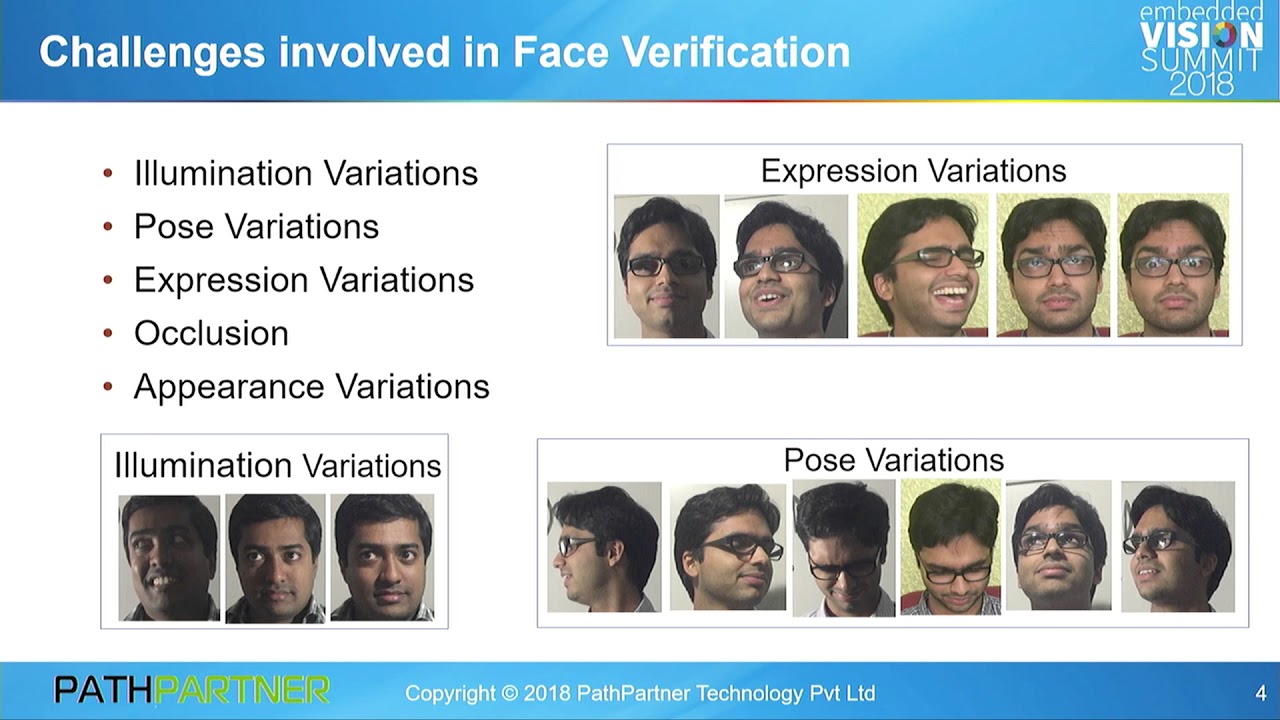
Показать описание
Praveen G.B., Technical Lead at PathPartner Technology, presents the "Creating a Computationally Efficient Embedded CNN Face Recognizer" tutorial at the May 2018 Embedded Vision Summit.
Face recognition systems have made great progress thanks to availability of data, deep learning algorithms and better image sensors. Face recognition systems should be tolerant of variations in illumination, pose and occlusions, and should be scalable to large numbers of users with minimal need for capturing images during registration. Machine learning approaches are limited by their scalability. Existing deep learning approaches make use of either "too-deep" networks with increased computational complexity or customized layers that require large model files.
In this talk, Praveen explores low-to-high complexity CNN architectures for face recognition and shows that with the right combination of training data and cost functions, you can indeed train a low-complexity CNN architecture (an AlexNet-like model, for example) that can achieve reasonably good accuracy compared with more-complex networks. He then explores system-level algorithmic customizations that will enable you to create a robust real-time embedded face recognition system using low-complexity CNN architectures.